Traffic flow forecasting method optimizing support vector regression by mixed artificial fish swarm algorithm
An artificial fish swarm algorithm and support vector regression technology, applied in traffic flow detection, calculation, special data processing applications, etc., can solve problems such as the inability to select optimal regression parameters, and the inability to obtain high prediction accuracy for traffic flow prediction applications.
- Summary
- Abstract
- Description
- Claims
- Application Information
AI Technical Summary
Problems solved by technology
Method used
Image
Examples
specific Embodiment approach
[0047] The prediction accuracy of the method proposed in the present invention and the established traffic flow model is illustrated by examples. The example uses the actual traffic flow data collected by the California Highway Performance Evaluation System (PeMS) as the source of experimental data, and the data sample time interval is 5 minutes. The experiment selects the traffic flow from 6:00 am to 10:00 am in a day as the experimental data set. The experimental data uses the data of five sites in the context of working days. The training set of each site has 576 data, and the 48 data of the 25th day are predicted. The example uses historical time series traffic flow data to predict future traffic flow. let x i (t) is the traffic flow at time t on road section i, and the experiment uses the traffic flow x of the first five time periods i (t-5),x i (t-4),x i (t-3),x i (t-2) and x i (t-1) as input, x i (t) as the predicted output of the model.
[0048] The initial pa...
PUM
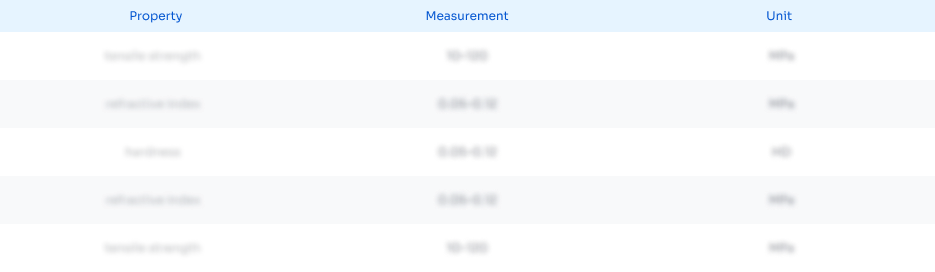
Abstract
Description
Claims
Application Information
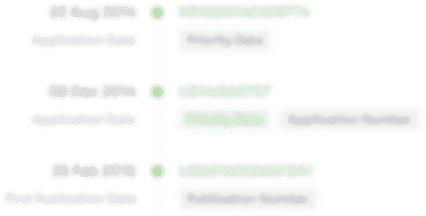
- Generate Ideas
- Intellectual Property
- Life Sciences
- Materials
- Tech Scout
- Unparalleled Data Quality
- Higher Quality Content
- 60% Fewer Hallucinations
Browse by: Latest US Patents, China's latest patents, Technical Efficacy Thesaurus, Application Domain, Technology Topic, Popular Technical Reports.
© 2025 PatSnap. All rights reserved.Legal|Privacy policy|Modern Slavery Act Transparency Statement|Sitemap|About US| Contact US: help@patsnap.com