Rolling bearing fault classifying method based on FOA-MKSVM (fruit fly optimization algorithm-multiple kernel support vector machine)
A rolling bearing and fault classification technology, applied in special data processing applications, instruments, electrical digital data processing, etc., can solve the problems of not constructing multi-core kernel functions, unbalanced state data, etc., and achieve good learning ability and generalization ability, initialization The effect of few parameters and simple parameter setting
- Summary
- Abstract
- Description
- Claims
- Application Information
AI Technical Summary
Problems solved by technology
Method used
Image
Examples
Embodiment Construction
[0042] The implementation process of the rolling bearing fault classification method based on FOA-MKSVM described in this embodiment is as follows:
[0043] Step 1. Use EEMD to combine SVD to perform feature extraction on the vibration signals of the normal state, inner ring fault state, outer ring fault state, and rolling element fault state of the rolling bearing to obtain the feature set of each state; two-thirds of the feature set are used as training features set, and one-third as the test feature set;
[0044] Step 2. Construct a multi-core kernel function to make the support vector machine multi-core, and obtain the multi-core support vector machine MKSVM:
[0045] Multiple different kernel functions are weighted and summed to construct a multi-kernel function K that adapts to different sample inputs of rolling bearing vibration signals mix (x i ,x j ):
[0046] k mix ( x i ...
PUM
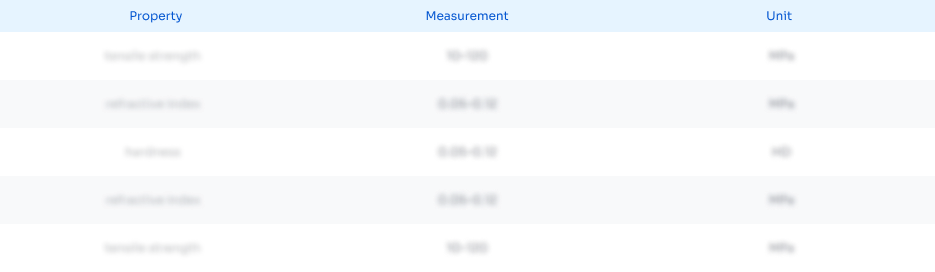
Abstract
Description
Claims
Application Information
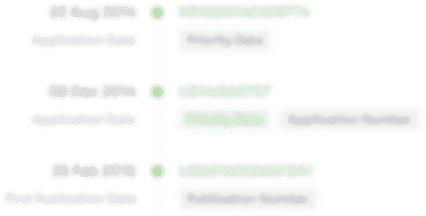
- R&D Engineer
- R&D Manager
- IP Professional
- Industry Leading Data Capabilities
- Powerful AI technology
- Patent DNA Extraction
Browse by: Latest US Patents, China's latest patents, Technical Efficacy Thesaurus, Application Domain, Technology Topic, Popular Technical Reports.
© 2024 PatSnap. All rights reserved.Legal|Privacy policy|Modern Slavery Act Transparency Statement|Sitemap|About US| Contact US: help@patsnap.com