Hyper-spectral classification method based on low-order mutual information and spectral context band selection
A technology of band selection and mutual information, applied in the field of hyperspectral image classification, it can solve the problems of ignoring correlation, high time complexity, poor classification accuracy, etc., so as to overcome the high time complexity, narrow the search range, and widen the application. Foreground effect
- Summary
- Abstract
- Description
- Claims
- Application Information
AI Technical Summary
Problems solved by technology
Method used
Image
Examples
Embodiment Construction
[0023] refer to figure 1 , the implementation steps of the present invention are as follows:
[0024] Step 1, input a hyperspectral image, obtain training samples and test samples.
[0025] input hyperspectral image The image contains l spectral bands and n samples;
[0026] Randomly select 20% samples from these n samples to form the training sample set Use the remaining samples to form the test sample set Among them, pp and qq represent the number of training and testing samples respectively, satisfying pp+qq=n.
[0027] Step 2, for the training sample set X pp and the test sample set X qq Perform column normalization operations respectively to obtain the training sample set X after column normalization p and the test sample set X q .
[0028] Step 3, calculate the training sample set X after column normalization p The self-information and regularized mutual information of the sample in the sample, remove the noise band, and get the label set of the noise band {...
PUM
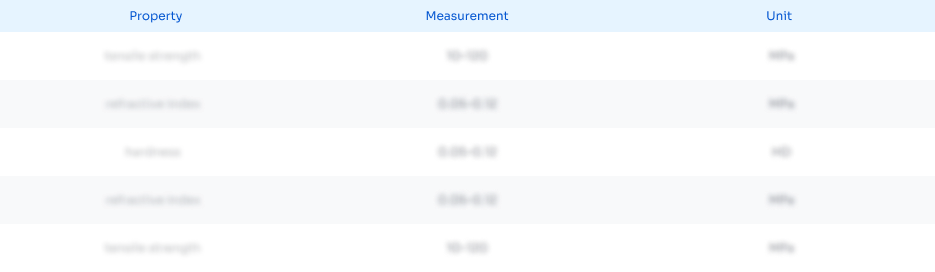
Abstract
Description
Claims
Application Information
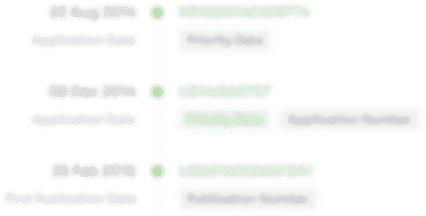
- R&D Engineer
- R&D Manager
- IP Professional
- Industry Leading Data Capabilities
- Powerful AI technology
- Patent DNA Extraction
Browse by: Latest US Patents, China's latest patents, Technical Efficacy Thesaurus, Application Domain, Technology Topic.
© 2024 PatSnap. All rights reserved.Legal|Privacy policy|Modern Slavery Act Transparency Statement|Sitemap