Bearing fault diagnosis method based on symbolic probabilistic finite state machine
A finite state machine and fault diagnosis technology, applied in the direction of mechanical bearing testing, etc., can solve the problems of KNN classification algorithm efficiency reduction, time increase, learning to obtain classifiers, etc., to improve computing efficiency and fault classification effect, improve stability and performance. The effect of practicality
- Summary
- Abstract
- Description
- Claims
- Application Information
AI Technical Summary
Problems solved by technology
Method used
Image
Examples
Embodiment Construction
[0024] The technical solutions of the invention will be described in detail below in conjunction with the accompanying drawings and examples, but the protection scope of the present invention is not limited to the examples.
[0025] Such as figure 1 As shown, the present invention provides a bearing fault diagnosis method based on a symbolic probability finite state machine, the method comprising the following steps:
[0026] (1) Use the acceleration sensor to collect a large number of bearing signals of known fault categories, including 30 groups of normal bearing vibration signals, 30 groups of 0.18mm inner ring faults, 30 groups of 0.36mm inner ring faults and 30 0.54mm inner ring faults Group. The bearing vibration signals of different fault categories are marked according to the category, and there are 4 different types of bearing signals, which are respectively marked as c 1 , c 2 , c 3 , c 4 , the number of samples for each type of bearing signal is 30, and there a...
PUM
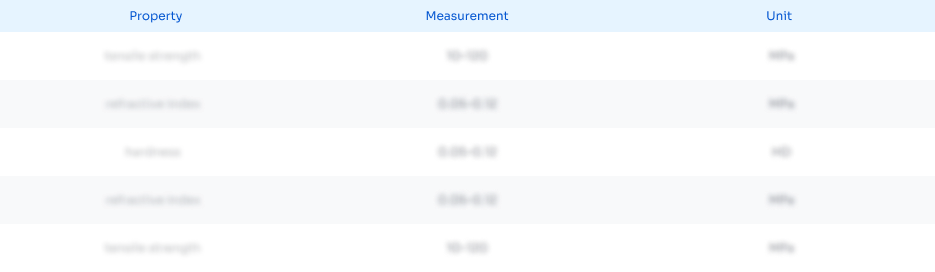
Abstract
Description
Claims
Application Information
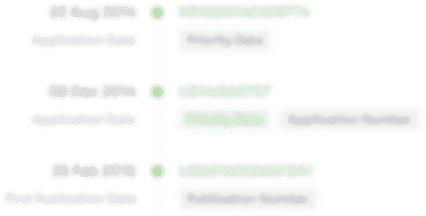
- R&D
- Intellectual Property
- Life Sciences
- Materials
- Tech Scout
- Unparalleled Data Quality
- Higher Quality Content
- 60% Fewer Hallucinations
Browse by: Latest US Patents, China's latest patents, Technical Efficacy Thesaurus, Application Domain, Technology Topic, Popular Technical Reports.
© 2025 PatSnap. All rights reserved.Legal|Privacy policy|Modern Slavery Act Transparency Statement|Sitemap|About US| Contact US: help@patsnap.com