Biological age step-by-step predication method based on support vector machine
A technology of support vector machine and prediction method, which is applied to computer parts, instruments, characters and pattern recognition, etc. It can solve the problems of cumbersome and complicated methods, low prediction efficiency and low accuracy, and achieves high classification accuracy and wide promotion. Application ability, the effect of improving accuracy
- Summary
- Abstract
- Description
- Claims
- Application Information
AI Technical Summary
Problems solved by technology
Method used
Image
Examples
specific Embodiment approach 1
[0029] Specific implementation mode one: combine figure 1 Description of this embodiment, a step-by-step prediction method of biological age based on support vector machine, is characterized in that, a step-by-step prediction method of biological age based on support vector machine is specifically carried out according to the following steps:
[0030] Step 1. Organize the physical property data of the biological samples obtained in the experiment to make a biological age data set (in the form of MS Excel, Notepad or ASCII coded file);
[0031] Classify the detected physical attribute data of biological samples, and store the different physical attribute data of each biological sample in a row in the matrix, that is, the different physical attribute data of a biological sample correspond to a row vector, and different biological samples are put together to form a matrix;
[0032] Wherein, the physical attribute data includes gender, length, diameter, height, total weight, weig...
specific Embodiment approach 2
[0045] Specific embodiment 2: The difference between this embodiment and specific embodiment 1 is that the combined training set obtained according to step 3 in step 4 uses a support vector machine classifier for training to generate a corresponding support vector machine model; specifically The process is:
[0046] Use the support vector machine classifier to train the merged training set obtained in step 3, and before generating the corresponding merged support vector machine model, first normalize the merged training set data, and map all the data To the pre-set value range, and then use the same mapping method to process the test set data;
[0047] The role of the normalization algorithm is: 1. Each attribute in the data set has an actual physical background, so their units and ranges are different. Normalization can eliminate the influence of units or orders of magnitude, and map all data to a predetermined range, which facilitates subsequent data processing; 2. Normaliz...
specific Embodiment approach 3
[0087]Specific embodiment three: the difference between this embodiment and specific embodiment one or two is that in the step five, the parameter optimization algorithm is used to optimize the penalty parameter C and the parameter γ in the RBF kernel function during the establishment of the support vector machine model, Establish the optimal support vector machine model according to the optimization results; the specific process is:
[0088] Parameter optimization algorithms include grid optimization algorithm, genetic algorithm and particle swarm optimization algorithm;
[0089] Grid search algorithm:
[0090] (1) Use the grid search method to find the optimal combination of the penalty parameter C of the optimal support vector machine model and the parameter γ of the support vector machine RBF kernel function;
[0091] The search is a two-step process:
[0092] The first step is a rough search, and the second step is a fine search;
[0093] In the first step of the searc...
PUM
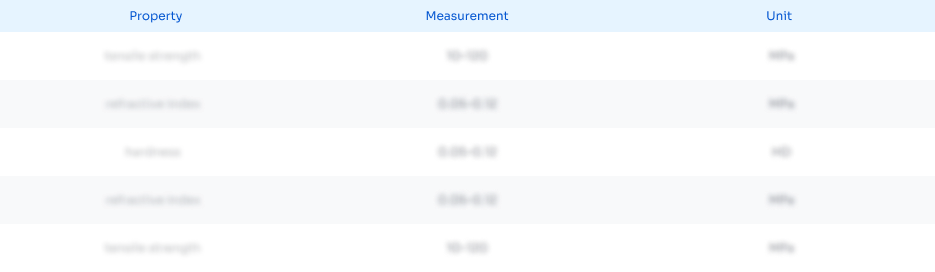
Abstract
Description
Claims
Application Information
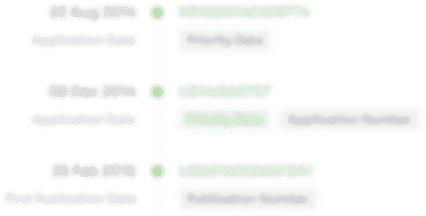
- R&D
- Intellectual Property
- Life Sciences
- Materials
- Tech Scout
- Unparalleled Data Quality
- Higher Quality Content
- 60% Fewer Hallucinations
Browse by: Latest US Patents, China's latest patents, Technical Efficacy Thesaurus, Application Domain, Technology Topic, Popular Technical Reports.
© 2025 PatSnap. All rights reserved.Legal|Privacy policy|Modern Slavery Act Transparency Statement|Sitemap|About US| Contact US: help@patsnap.com