Regularization-based RBF network multi-label classification method
A technology of RBF network and classification method, which is applied in the field of regularized RBF network multi-label classification, which can solve the problems of reducing the generalization performance of classification methods, the problem of not being able to classify multiple labels, and the classification results that cannot be obtained by classification methods.
- Summary
- Abstract
- Description
- Claims
- Application Information
AI Technical Summary
Problems solved by technology
Method used
Image
Examples
Embodiment
[0112] This example takes the Yeast dataset as an example. The dataset belongs to the field of biology and includes 1500 training samples and 917 test samples. The training samples are used to train a multi-label classification system, and the test samples are used as samples with unknown labels for label prediction. The Yeast dataset has 103-dimensional features and 14 labels, with an average number of labels of 4.24 and a label density of 0.303.
[0113] Regularized RBF network multi-label classification method, such as figure 1 As shown, using a training data set containing 1500 samples to construct an RBF network includes 3 steps, and the specific process is as follows:
[0114] Step 1: The network input layer includes 103 nodes;
[0115] Step 2: Label space with dimension 14 for the dataset for each label in , find the positive sample set in the training data set For clustering, the specific steps are:
[0116] 1) Label collection label y in 1 , find out the po...
PUM
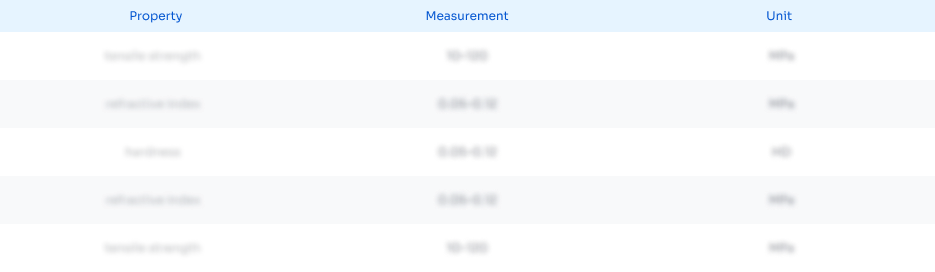
Abstract
Description
Claims
Application Information
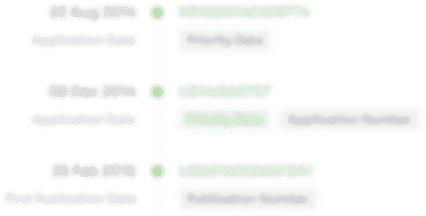
- R&D
- Intellectual Property
- Life Sciences
- Materials
- Tech Scout
- Unparalleled Data Quality
- Higher Quality Content
- 60% Fewer Hallucinations
Browse by: Latest US Patents, China's latest patents, Technical Efficacy Thesaurus, Application Domain, Technology Topic, Popular Technical Reports.
© 2025 PatSnap. All rights reserved.Legal|Privacy policy|Modern Slavery Act Transparency Statement|Sitemap|About US| Contact US: help@patsnap.com