Multiple observed value vector sparsity self-adaptive compressed sampling matching pursuit method
A compression sampling and matching pursuit technology, applied in the field of information and communication
- Summary
- Abstract
- Description
- Claims
- Application Information
AI Technical Summary
Problems solved by technology
Method used
Image
Examples
specific Embodiment approach 1
[0106] Specific implementation mode 1. Combination Image 6 Describe this specific implementation method, multi-observation value vector sparsity adaptive compression sampling matching tracking method, the specific process is: input observation matrix A and frame matrix V, set an appropriate residual threshold θ according to the size of the signal-to-noise ratio, and combine signals according to The approximate range of sparsity sets an appropriate stage number threshold σ.
[0107] initialization order support set Residual R=V, support set candidate set According to the approximate range of signal joint sparsity, set the appropriate number of stages stage and step size step. Computes the 2-norm of each atom (column vector) in the observation matrix. Repeat the following steps until the iteration stop condition is met:
[0108] Use the formula:
[0109] s=stage×step(1)
[0110] Compute the estimated sparsity s for each iteration. Multiply the residual matrix R (initia...
PUM
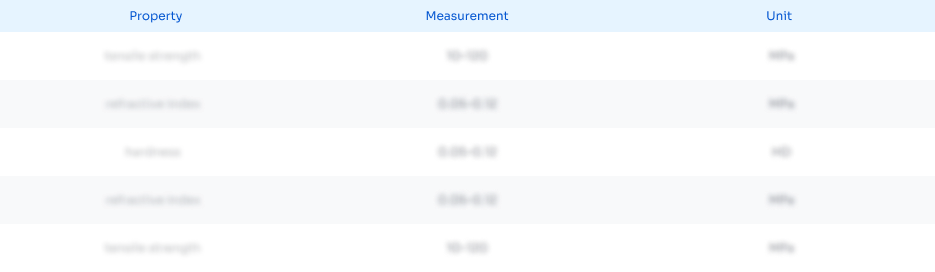
Abstract
Description
Claims
Application Information
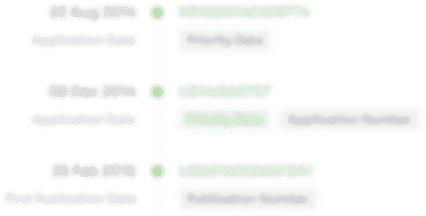
- R&D Engineer
- R&D Manager
- IP Professional
- Industry Leading Data Capabilities
- Powerful AI technology
- Patent DNA Extraction
Browse by: Latest US Patents, China's latest patents, Technical Efficacy Thesaurus, Application Domain, Technology Topic, Popular Technical Reports.
© 2024 PatSnap. All rights reserved.Legal|Privacy policy|Modern Slavery Act Transparency Statement|Sitemap|About US| Contact US: help@patsnap.com