Multi-modal process fault detection method based on local neighbor standardization matrix
A fault detection, multi-modal technology, applied in program control, electrical test/monitoring, test/monitoring control system, etc., can solve the problem of final fault diagnosis accuracy, different batch production cycles, pivot direction deviation, etc. problems, to achieve the effect of highlighting differences, improving accuracy, and reducing the degree of deviation
- Summary
- Abstract
- Description
- Claims
- Application Information
AI Technical Summary
Problems solved by technology
Method used
Image
Examples
Embodiment Construction
[0017] The present invention will be described in detail below in conjunction with embodiments.
[0018] The invention uses a local weighting algorithm to preprocess the unequal-length batch process, and process the unequal-length batch into an equal-length batch. On the basis of preprocessing, the normalized matrix of main and local neighbors is constructed on the training set of equal length, the K-means algorithm is used for modal clustering, the local outlier factor method is used to eliminate outlier samples of each mode, and then the outlier samples of each mode are eliminated. Establish MPCA model for intermittent process fault detection. This technology solves the fault detection problem of unequal-length batches and multi-modal processes in intermittent production processes. In order to better perform modal clustering, it is necessary to find statistical features that highlight the characteristics of each modal. The present invention proposes to use the local nearest n...
PUM
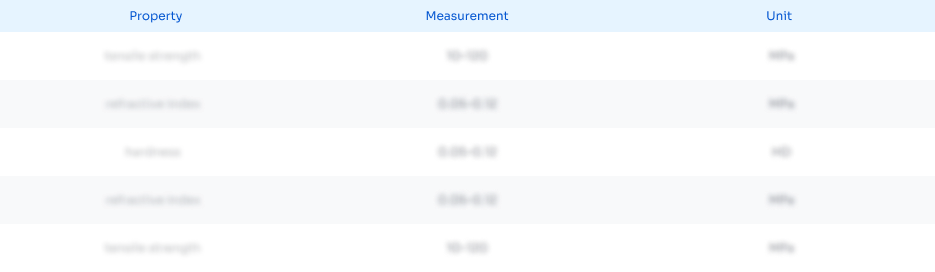
Abstract
Description
Claims
Application Information
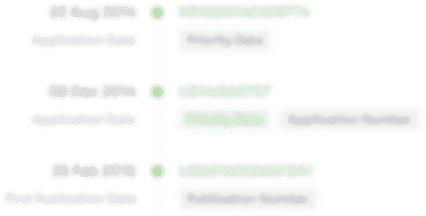
- Generate Ideas
- Intellectual Property
- Life Sciences
- Materials
- Tech Scout
- Unparalleled Data Quality
- Higher Quality Content
- 60% Fewer Hallucinations
Browse by: Latest US Patents, China's latest patents, Technical Efficacy Thesaurus, Application Domain, Technology Topic, Popular Technical Reports.
© 2025 PatSnap. All rights reserved.Legal|Privacy policy|Modern Slavery Act Transparency Statement|Sitemap|About US| Contact US: help@patsnap.com