Based on gb(2d) 2 Face recognition method of pcanet deep convolution model
An identity recognition and deep convolution technology, applied in character and pattern recognition, neural learning methods, biological neural network models, etc. Effect
- Summary
- Abstract
- Description
- Claims
- Application Information
AI Technical Summary
Problems solved by technology
Method used
Image
Examples
Embodiment Construction
[0034] In order to better illustrate the purpose, specific steps and characteristics of the present invention, the present invention will be further described in detail below in conjunction with the accompanying drawings, taking the AR face library [5] as an example:
[0035] The present invention proposes a method based on GB(2D) 2 Face recognition method of PCANet deep convolution model, where GB(2D) 2 PCANet depth convolution model such as figure 1 shown. GB(2D) 2 PCANet consists of two feature extraction layers and a nonlinear output layer. The convolution filter of the feature extraction layer is composed of Gabor and (2D) 2 PCA learning is used to convolve the original input image to extract features, and the nonlinear output layer includes binary hash and local histogram calculation operations, which are used to further calculate the final features.
[0036] The present invention proposes a method based on GB(2D) 2 Face recognition method of PCANet deep convolution...
PUM
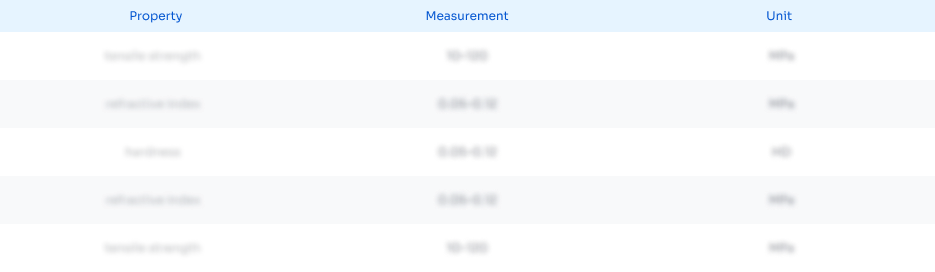
Abstract
Description
Claims
Application Information
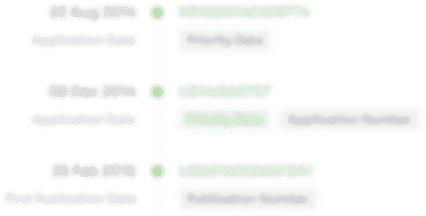
- R&D
- Intellectual Property
- Life Sciences
- Materials
- Tech Scout
- Unparalleled Data Quality
- Higher Quality Content
- 60% Fewer Hallucinations
Browse by: Latest US Patents, China's latest patents, Technical Efficacy Thesaurus, Application Domain, Technology Topic, Popular Technical Reports.
© 2025 PatSnap. All rights reserved.Legal|Privacy policy|Modern Slavery Act Transparency Statement|Sitemap|About US| Contact US: help@patsnap.com