Sparse dictionary-based wireless sensor network missing data reconstruction method
A wireless sensor, missing data technology, applied in the direction of transmission data organization to avoid errors, digital transmission systems, transmission systems, etc. The effect of high precision and reduction of reconstruction errors
- Summary
- Abstract
- Description
- Claims
- Application Information
AI Technical Summary
Problems solved by technology
Method used
Image
Examples
Embodiment Construction
[0035] In order to make the objectives, technical solutions and advantages of the present invention clearer, the present invention will be further described in detail below with reference to the embodiments. It should be understood that the specific embodiments described herein are only used to explain the present invention, but not to limit the present invention.
[0036] like figure 1 As shown, a method for reconstructing missing data in wireless sensor networks based on sparse dictionary includes the following steps:
[0037] 1) Determine the total number N of data frames to be reconstructed according to the missing data; in the historical data, select M data frames as training data, where M is an integer greater than K;
[0038] 2) Call the K-SVD algorithm to obtain a dictionary D of size K;
[0039] 3) For dictionary D, use L 1 Norm minimization algorithm to obtain each dictionary atom d i Corresponding sparse coefficient α i ;
[0040] 4) According to the calculati...
PUM
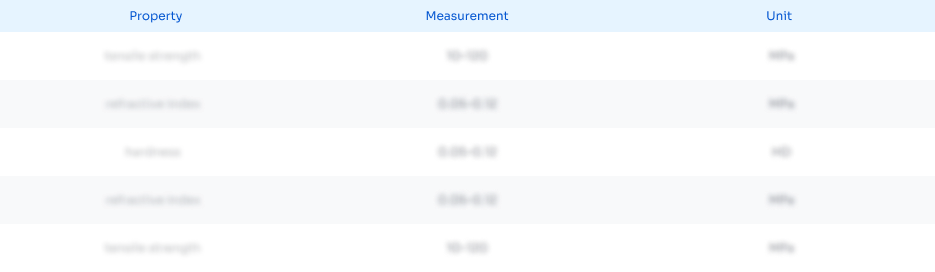
Abstract
Description
Claims
Application Information
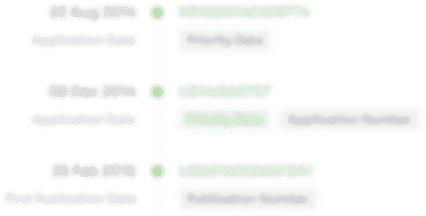
- R&D
- Intellectual Property
- Life Sciences
- Materials
- Tech Scout
- Unparalleled Data Quality
- Higher Quality Content
- 60% Fewer Hallucinations
Browse by: Latest US Patents, China's latest patents, Technical Efficacy Thesaurus, Application Domain, Technology Topic, Popular Technical Reports.
© 2025 PatSnap. All rights reserved.Legal|Privacy policy|Modern Slavery Act Transparency Statement|Sitemap|About US| Contact US: help@patsnap.com