A Human Behavior Recognition Method Based on Sparse Low Rank
A recognition method and sparse technology, which can be used in character and pattern recognition, instruments, computing, etc., and can solve problems such as information loss, high computational complexity, and human behavior occlusion.
- Summary
- Abstract
- Description
- Claims
- Application Information
AI Technical Summary
Problems solved by technology
Method used
Image
Examples
Embodiment Construction
[0052] Implementation language: Matlab
[0053] Hardware platform: Intel i3 2120+4G DDR RAM
[0054] The method of the invention is verified through an intuitive and effective algorithm on Matlab.
[0055] The bag-of-words method, low-rank method and the method described in this patent are tested by collecting pedestrian activities in the school square. Pedestrian activities mainly include: bending, falling, clapping, waving, running, squatting, and walking 7 behaviors, the test results ,like Figure 4 shown. In contrast, using the method described in this patent has achieved better recognition results. Using the bag-of-words method ( Figure 4 A) The recognition effect is significantly lower than the low-rank representation method and the method described in this patent, and the low-rank representation method is basically the same as the low-rank sparse representation method in bending and falling actions, but slightly lower than the low-rank representation method in othe...
PUM
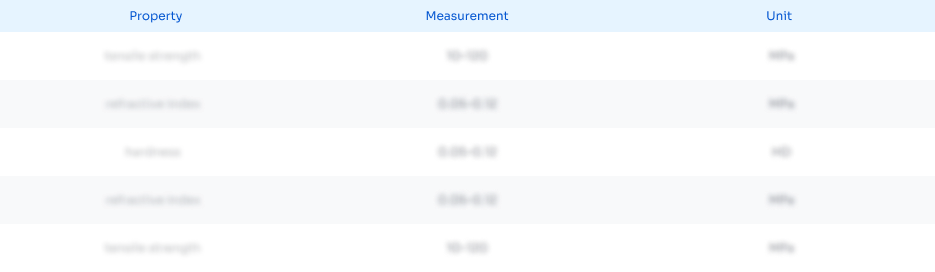
Abstract
Description
Claims
Application Information
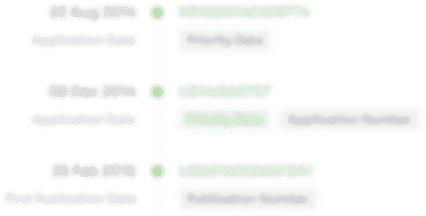
- R&D Engineer
- R&D Manager
- IP Professional
- Industry Leading Data Capabilities
- Powerful AI technology
- Patent DNA Extraction
Browse by: Latest US Patents, China's latest patents, Technical Efficacy Thesaurus, Application Domain, Technology Topic, Popular Technical Reports.
© 2024 PatSnap. All rights reserved.Legal|Privacy policy|Modern Slavery Act Transparency Statement|Sitemap|About US| Contact US: help@patsnap.com