CNN (convolutional neural network)-based fMRI (functional magnetic resonance imaging) visual function data object extraction method
A technology of visual function data and target extraction, applied in image data processing, instruments, computing and other directions, can solve problems such as lack of research results, and achieve the effect of improving analytical ability and accuracy
- Summary
- Abstract
- Description
- Claims
- Application Information
AI Technical Summary
Problems solved by technology
Method used
Image
Examples
Embodiment 1
[0025] Embodiment one, see figure 1 As shown, a CNN-based fMRI visual function data target extraction method includes the following steps:
[0026] Step 1. Collect fMRI visual function data of subjects stimulated by natural images in complex scenes, train a deep convolutional neural network model from stimulus images to fMRI visual function data, and a linear model from fMRI visual function data to target categories Mapping model, deep convolutional neural network model includes convolution layer, rectified linear unit layer, maximum pooling layer and fully connected layer;
[0027] Step 2. Add a feedback layer to the deep convolutional neural network model to obtain a convolutional neurofeedback model. The convolutional neurofeedback model is combined with the linear mapping model obtained in step 1 to obtain a category scoring map;
[0028] Step 3. Analyze the fMRI visual function data of the subjects watching the brand-new test images, and use the category score mapping to...
Embodiment 2
[0029] Embodiment two, see figure 1 As shown, a CNN-based fMRI visual function data target extraction method includes the following steps:
[0030] Step 1. Collect fMRI visual function data of subjects stimulated by natural images in complex scenes, train a deep convolutional neural network model from stimulus images to fMRI visual function data, and a linear model from fMRI visual function data to target categories Mapping model, deep convolutional neural network model includes convolution layer, rectified linear unit layer, maximum pooling layer and fully connected layer;
[0031] Step 2. Add a feedback layer to the deep convolutional neural network model to obtain a convolutional neurofeedback model. The convolutional neurofeedback model is compounded with the linear mapping model obtained in step 1 to obtain a category scoring map, which specifically includes the following steps:
[0032] Step 2.1 Stack a feedback layer after each rectified linear unit layer in the deep c...
PUM
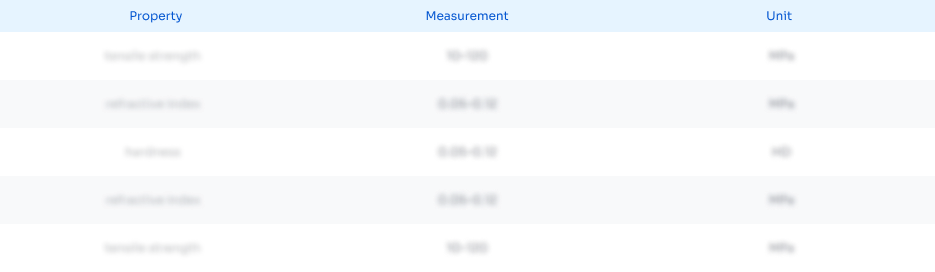
Abstract
Description
Claims
Application Information
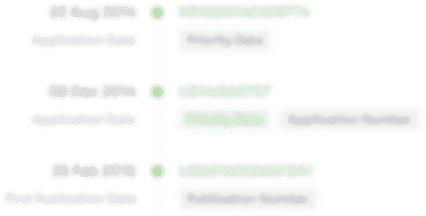
- R&D Engineer
- R&D Manager
- IP Professional
- Industry Leading Data Capabilities
- Powerful AI technology
- Patent DNA Extraction
Browse by: Latest US Patents, China's latest patents, Technical Efficacy Thesaurus, Application Domain, Technology Topic, Popular Technical Reports.
© 2024 PatSnap. All rights reserved.Legal|Privacy policy|Modern Slavery Act Transparency Statement|Sitemap|About US| Contact US: help@patsnap.com