Pedestrian recognition method of camera network based on multi-level depth feature fusion
A camera network and depth feature technology, which is applied in the field of computer vision monitoring, can solve the problems of difficulty in extracting robust depth features of pedestrian images, inability to fully utilize depth features, and insufficient depth network training to achieve the effect of improving accuracy.
- Summary
- Abstract
- Description
- Claims
- Application Information
AI Technical Summary
Problems solved by technology
Method used
Image
Examples
Embodiment Construction
[0020] In order to describe the present invention more specifically, the technical solutions of the present invention will be described in detail below in conjunction with the accompanying drawings and specific embodiments.
[0021] The method of the invention includes two parts: the construction of a deep network model on the pedestrian database and the extraction and fusion of multi-level deep features. We transfer the pre-trained network parameters to the pedestrian database to help the learning of the target network on the pedestrian database, use the target network to extract multiple levels of depth features of pedestrian samples, and then use the depth features of different levels to construct multiple sets of binary classification SVM classifiers, and linearly weight the decision values of these two classifiers to obtain the final classification results. Below in conjunction with accompanying drawing, the inventive method will be further described:
[0022] figure ...
PUM
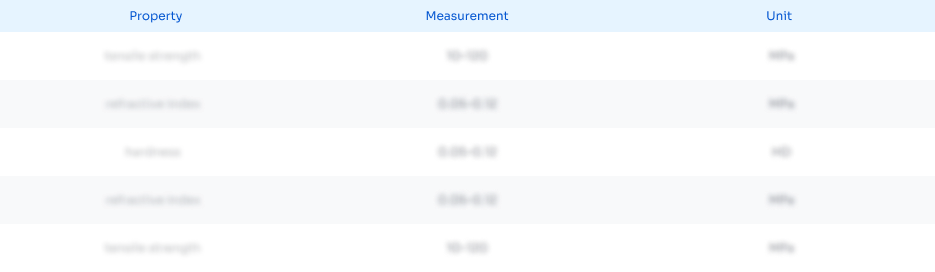
Abstract
Description
Claims
Application Information
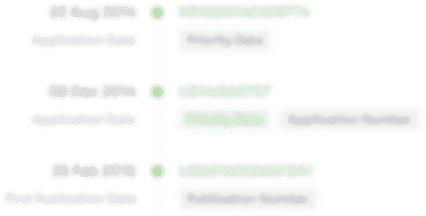
- R&D Engineer
- R&D Manager
- IP Professional
- Industry Leading Data Capabilities
- Powerful AI technology
- Patent DNA Extraction
Browse by: Latest US Patents, China's latest patents, Technical Efficacy Thesaurus, Application Domain, Technology Topic, Popular Technical Reports.
© 2024 PatSnap. All rights reserved.Legal|Privacy policy|Modern Slavery Act Transparency Statement|Sitemap|About US| Contact US: help@patsnap.com