Customer segmentation system based on improved k-means and neural network clustering
A neural network and neural network training technology, applied in the field of data mining, can solve the problems of low clustering stability and inability to effectively avoid contingency, and achieve the effects of improving clustering stability, avoiding contingency, and improving accuracy
- Summary
- Abstract
- Description
- Claims
- Application Information
AI Technical Summary
Problems solved by technology
Method used
Image
Examples
Embodiment 1
[0032] see figure 1 , figure 2 , the customer segmentation system based on improved k-means and neural network clustering of the present embodiment includes a bank customer data acquisition module 1, a sample data extraction module 2, a clustering processing module 3, a neural network training module 4, and a customer category detail Sub-module 5, the bank customer data collection module 1 is used to collect bank customer data, and bank customer data is stored in the bank network database; the sample data extraction module 2 is used to extract bank customer data from the bank network database Random sampling, extracting one-third of the data as sample data; the clustering processing module 3 is used to cluster each sample of the sample data by using the improved k-means clustering method, and output the clustering result; the neural network The training module 4 is used to use the clustering result as a training sample, and uses a neural network to calculate the weight of ea...
Embodiment 2
[0049] see figure 1 , figure 2 , the customer segmentation system based on improved k-means and neural network clustering of the present embodiment includes a bank customer data acquisition module 1, a sample data extraction module 2, a clustering processing module 3, a neural network training module 4, and a customer category detail Sub-module 5, the bank customer data collection module 1 is used to collect bank customer data, and bank customer data is stored in the bank network database; the sample data extraction module 2 is used to extract bank customer data from the bank network database Random sampling, extracting one-third of the data as sample data; the clustering processing module 3 is used to cluster each sample of the sample data by using the improved k-means clustering method, and output the clustering result; the neural network The training module 4 is used to use the clustering result as a training sample, and uses a neural network to calculate the weight of ea...
Embodiment 3
[0066] see figure 1 , figure 2 , the customer segmentation system based on improved k-means and neural network clustering of the present embodiment includes a bank customer data acquisition module 1, a sample data extraction module 2, a clustering processing module 3, a neural network training module 4, and a customer category detail Sub-module 5, the bank customer data collection module 1 is used to collect bank customer data, and bank customer data is stored in the bank network database; the sample data extraction module 2 is used to extract bank customer data from the bank network database Random sampling, extracting one-third of the data as sample data; the clustering processing module 3 is used to cluster each sample of the sample data by using the improved k-means clustering method, and output the clustering result; the neural network The training module 4 is used to use the clustering result as a training sample, and uses a neural network to calculate the weight of ea...
PUM
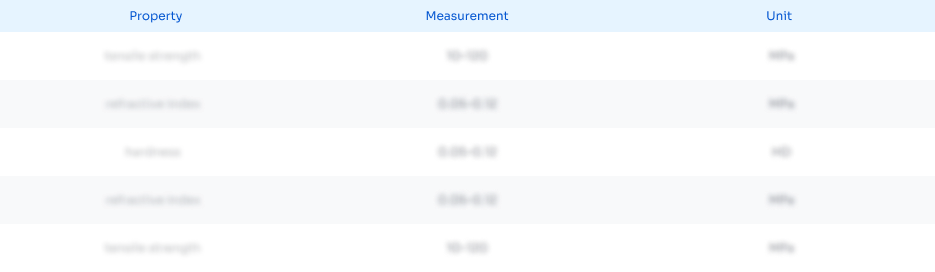
Abstract
Description
Claims
Application Information
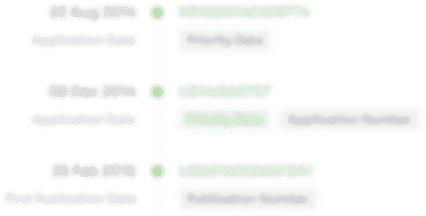
- R&D
- Intellectual Property
- Life Sciences
- Materials
- Tech Scout
- Unparalleled Data Quality
- Higher Quality Content
- 60% Fewer Hallucinations
Browse by: Latest US Patents, China's latest patents, Technical Efficacy Thesaurus, Application Domain, Technology Topic, Popular Technical Reports.
© 2025 PatSnap. All rights reserved.Legal|Privacy policy|Modern Slavery Act Transparency Statement|Sitemap|About US| Contact US: help@patsnap.com