Weighted sparse-based mixed denoising method
A mixed noise and sparse technology, applied in image data processing, instruments, character and pattern recognition, etc., can solve the problems of difficult removal of mixed noise, blurred edges of denoised images, smooth denoised images, etc., to improve visual effects, enhance Noise removal, the effect of effective noise removal
- Summary
- Abstract
- Description
- Claims
- Application Information
AI Technical Summary
Problems solved by technology
Method used
Image
Examples
Embodiment Construction
[0022] A mixed noise removal method based on weighted sparsity, such as figure 1 shown, including the following steps:
[0023] Step 1. Obtain a natural image in a standard image library, perform normalized preprocessing on the image, and then perform image segmentation.
[0024] Obtain natural images in the standard image library. The size of the images is all 512×512, and the gray value is between 0-255. In order to simplify the calculation amount, each image is normalized. After the normalization process, the The image is divided into blocks, and the size of each block is divided into 7×7 according to the present invention, so each image has 49 small blocks.
[0025] Step 2, add noise to each image, and use the method of variation and weight sparse coding to get the mixed noise removal model.
[0026] First, add noise to each image, let y∈R n is a noisy observation map, x∈R n is the denoising image, n is Gaussian white noise, then the mathematical formula of Gaussian wh...
PUM
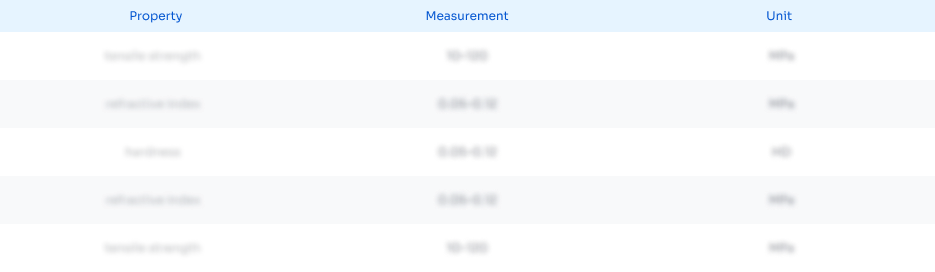
Abstract
Description
Claims
Application Information
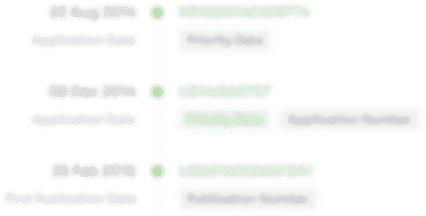
- R&D Engineer
- R&D Manager
- IP Professional
- Industry Leading Data Capabilities
- Powerful AI technology
- Patent DNA Extraction
Browse by: Latest US Patents, China's latest patents, Technical Efficacy Thesaurus, Application Domain, Technology Topic.
© 2024 PatSnap. All rights reserved.Legal|Privacy policy|Modern Slavery Act Transparency Statement|Sitemap