Dimension reduction clustering data analysis method
A data analysis and clustering technology, applied in the computer field, can solve problems such as lack of theoretical basis
- Summary
- Abstract
- Description
- Claims
- Application Information
AI Technical Summary
Problems solved by technology
Method used
Image
Examples
Embodiment 1
[0036] This embodiment provides a data analysis method for dimensionality reduction clustering, which includes:
[0037] S101. By generating sample data, perform dimensionless processing on the sample data to construct projection data required by the model.
[0038] In this embodiment, the projection data required by the model can be constructed by generating sample data. For example: According to the classification standard table, a certain amount of sample data is randomly generated within each level. Mark the influence index of the sample data as X ij (i=1,2,…,n; j=1,2,…m), where n is a natural number, indicating the number of samples; m is a natural number, indicating the number of variables, if the classification standard table is divided into 5 levels, To generate 100 samples in each level range, the number of samples n is 500.
[0039] Since the dimensions of the variables in the sample data are not the same or the value ranges are quite different, it is necessary to...
Embodiment 2
[0068] This example demonstrates the data analysis method of dimensionality reduction clustering through specific operations, that is, a water quality analysis method, see Table 1-1 below, which is the water quality monitoring result table of a certain reservoir in a certain month, first according to the "Surface Water "Environmental Quality Standards" Table 1-2 to evaluate this reservoir, the steps in Example 1 can be used.
[0069] Table 1-1 Water quality monitoring results of a certain reservoir in a certain month
[0070]
[0071] Table 1-2 Reservoir water quality evaluation standard table
[0072]
[0073] 30 samples were randomly generated within the scope of each water quality standard level, and the reservoir water quality evaluation standard is divided into 5 levels, so a total of 150 water quality samples were obtained. Based on the generated sample data at all levels, the water quality evaluation model of the reservoir based on the projection pursuit dynamic ...
PUM
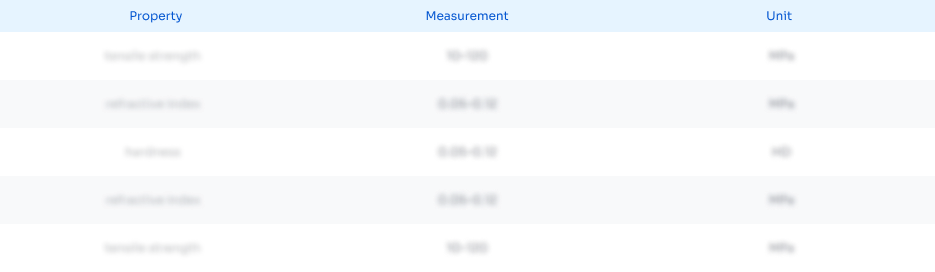
Abstract
Description
Claims
Application Information
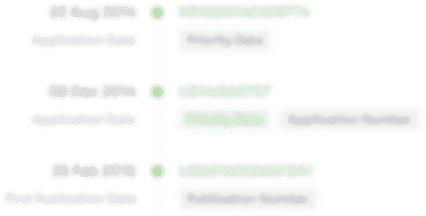
- R&D Engineer
- R&D Manager
- IP Professional
- Industry Leading Data Capabilities
- Powerful AI technology
- Patent DNA Extraction
Browse by: Latest US Patents, China's latest patents, Technical Efficacy Thesaurus, Application Domain, Technology Topic, Popular Technical Reports.
© 2024 PatSnap. All rights reserved.Legal|Privacy policy|Modern Slavery Act Transparency Statement|Sitemap|About US| Contact US: help@patsnap.com