Depth-learning-theory-based fault diagnosis method for rotating machinery
A technology for rotating machinery equipment and fault diagnosis, applied in neural learning methods, biological neural network models, etc., can solve the problem of not overcoming frequency ambiguity, not using temperature information in time domain, and not providing deep neural network self-learning and self-improvement. and other problems to achieve the effect of eliminating non-stationarity, accurate diagnosis and accurate diagnosis.
- Summary
- Abstract
- Description
- Claims
- Application Information
AI Technical Summary
Problems solved by technology
Method used
Image
Examples
Embodiment Construction
[0025] The specific implementation of the present invention will be described below by taking the bearing fault diagnosis of a certain type of wind turbine as an example in conjunction with the accompanying drawings.
[0026] 1. Construct a deep neural network DNN, including the type of deep neural network DNN, the number of layers, and the number of nodes in each layer;
[0027] The type of deep neural network described in further step 1 includes: autoencoder AutoEncoder, denoising autoencoder Denoising Autoencode, sparse coding Sparse coding, limit Boltzmann machine RestrictedBoltzmann Machine (RBM), deep belief network Deep Belief Networks , Convolutional Neural Networks;
[0028] According to the characteristics of vibration signals of mechanical faults, this embodiment selects DenoisingAutoencode with a 7-layer denoising automatic encoding machine, and the number of nodes in each layer is 2,097,170.
[0029] Because the environment of mechanical equipment is complex, the...
PUM
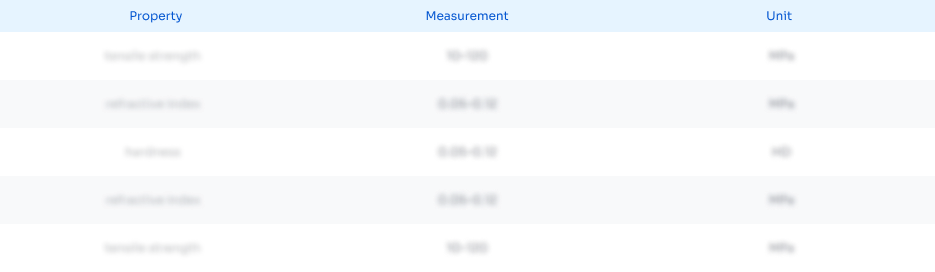
Abstract
Description
Claims
Application Information
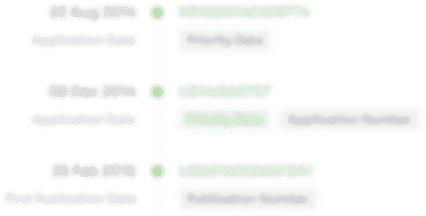
- R&D Engineer
- R&D Manager
- IP Professional
- Industry Leading Data Capabilities
- Powerful AI technology
- Patent DNA Extraction
Browse by: Latest US Patents, China's latest patents, Technical Efficacy Thesaurus, Application Domain, Technology Topic, Popular Technical Reports.
© 2024 PatSnap. All rights reserved.Legal|Privacy policy|Modern Slavery Act Transparency Statement|Sitemap|About US| Contact US: help@patsnap.com