Target tracking-before-detecting method based on Gaussian cardinalized probability hypothesis density filter
A technology of hypothetical density and target detection, applied in radio wave measurement systems, radio wave reflection/re-radiation, measurement devices, etc., can solve problems such as high complexity, large particle support set, and sampling exhaustion
- Summary
- Abstract
- Description
- Claims
- Application Information
AI Technical Summary
Problems solved by technology
Method used
Image
Examples
Embodiment Construction
[0040] refer to figure 1 , is a flow chart of a target tracking method before detection based on Gaussian particle potential probability assumption density filtering of the present invention; the target tracking method based on Gaussian particle potential probability assumption density filtering includes the following steps:
[0041] Step 1, initialization: let k represent time k, the initial value of k is 1, k∈{1,2,...,D}, D represents the maximum time set, and D is the movement time of observing each target; this In the embodiment, D=60 is set.
[0042] 0 time N 0 The motion state of a target is denoted as x 0 , set 0 time N 0 The motion state x of a target 0 The intensity at (i,j) is denoted as and abbreviated as z 0 ; Then calculate N at time 0 0 The motion state x of a target 0 Intensity at (i,j) condition 0 time N 0 The motion state x of a target 0 The posterior probability density p(x 0 |z 0 ), p(x 0 |z 0 )=N(x 0 μ 0 ,P 0 ), N(x 0 μ 0 ,P 0 ) means...
PUM
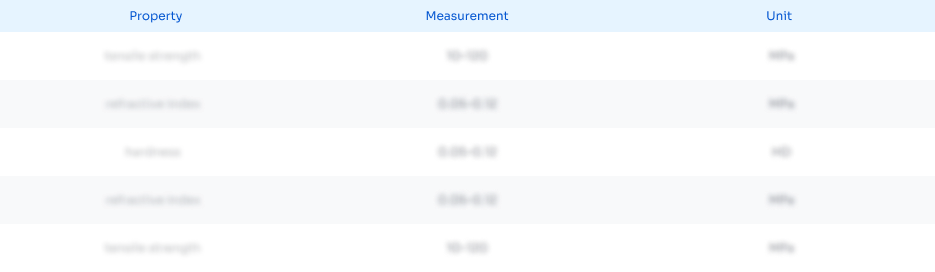
Abstract
Description
Claims
Application Information
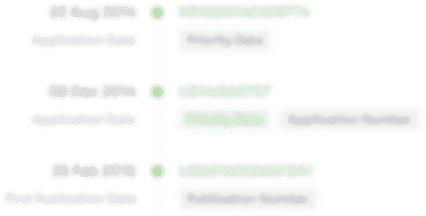
- R&D Engineer
- R&D Manager
- IP Professional
- Industry Leading Data Capabilities
- Powerful AI technology
- Patent DNA Extraction
Browse by: Latest US Patents, China's latest patents, Technical Efficacy Thesaurus, Application Domain, Technology Topic, Popular Technical Reports.
© 2024 PatSnap. All rights reserved.Legal|Privacy policy|Modern Slavery Act Transparency Statement|Sitemap|About US| Contact US: help@patsnap.com