Application of deep learning based on fuzzy processing in fault diagnosis of hydraulic equipment
A technology of deep learning and fuzzy processing, applied in fluid pressure actuation devices, mechanical equipment, fluid pressure actuation system testing, etc., can solve problems such as inapplicability to large-scale data processing, and achieve the effect of classification and diagnosis of equipment faults
- Summary
- Abstract
- Description
- Claims
- Application Information
AI Technical Summary
Problems solved by technology
Method used
Image
Examples
Embodiment Construction
[0098] An application of deep learning based on fuzzy processing in hydraulic equipment fault diagnosis, including the following steps:
[0099] (1) Introduce time labels and fuzzy weights to preprocess hydraulic equipment operation monitoring data, and divide them into training data sets and test data sets, and label the test data sets: mark the specific fault status of the equipment and divide them into two parts One part is used to train the ICM model, and the other part is used to verify the performance of the ICM model.
[0100] Data preprocessing includes the following steps:
[0101] 1) Sample feature selection and normalization: use the hydraulic equipment operation monitoring data of an iron and steel enterprise to establish an equipment monitoring data set, and select the operating status data of hydraulic equipment with time tags (monitoring data includes normal status data and fault status data). The hydraulic equipment is divided into two groups, A and B, and the...
PUM
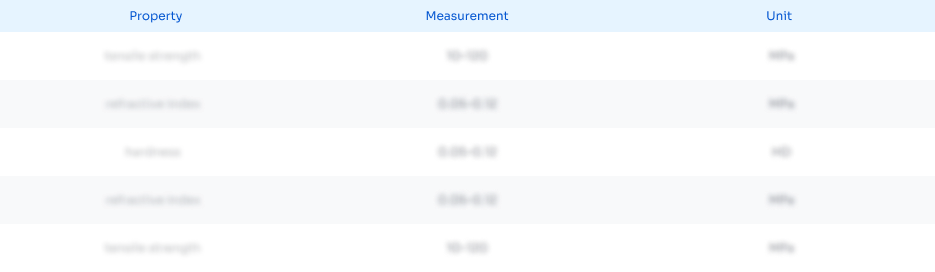
Abstract
Description
Claims
Application Information
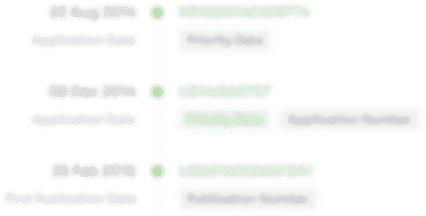
- R&D Engineer
- R&D Manager
- IP Professional
- Industry Leading Data Capabilities
- Powerful AI technology
- Patent DNA Extraction
Browse by: Latest US Patents, China's latest patents, Technical Efficacy Thesaurus, Application Domain, Technology Topic, Popular Technical Reports.
© 2024 PatSnap. All rights reserved.Legal|Privacy policy|Modern Slavery Act Transparency Statement|Sitemap|About US| Contact US: help@patsnap.com