Recommended system and method with facing social network for context awareness based on tensor decomposition
A tensor decomposition and social network technology, applied in the field of context-aware recommendation, can solve problems such as cold start, increased computational complexity, and limited feature extraction capabilities, and achieve the effect of improving accuracy
- Summary
- Abstract
- Description
- Claims
- Application Information
AI Technical Summary
Problems solved by technology
Method used
Image
Examples
Embodiment Construction
[0017] In order to make the objectives, technical solutions and advantages of the present invention clearer, the specific implementation of the invention will be further elaborated below with reference to the accompanying drawings of the specification.
[0018] Such as figure 1 It is the overall flow chart of the present invention, including data acquisition, filling sparse scoring matrix, filling user relationship matrix, and optimizing dense scoring matrix four modules. Among them, data acquisition can be directly downloaded from a web-based research recommendation system or use mature social Platform API acquisition.
[0019] The implementation of the present invention mainly includes the following steps:
[0020] S1: Obtain data sources and obtain user information collection, watch list collection, item information collection, and context information collection.
[0021] S2: Construct a user-item-context rating matrix based on user, item, and contextual information. On the basis o...
PUM
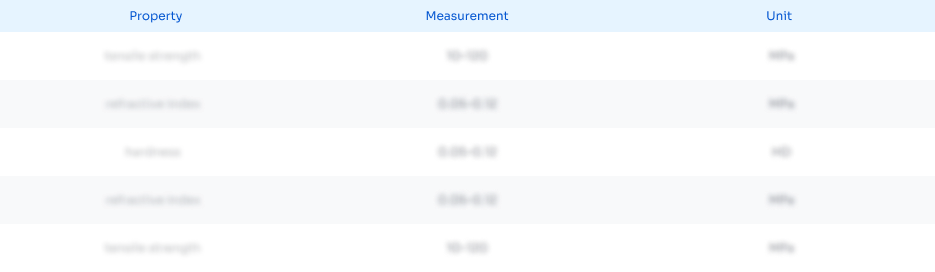
Abstract
Description
Claims
Application Information
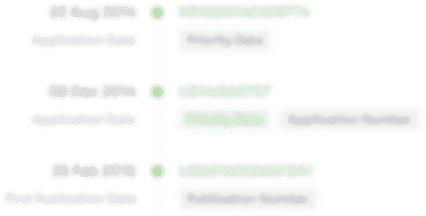
- R&D Engineer
- R&D Manager
- IP Professional
- Industry Leading Data Capabilities
- Powerful AI technology
- Patent DNA Extraction
Browse by: Latest US Patents, China's latest patents, Technical Efficacy Thesaurus, Application Domain, Technology Topic.
© 2024 PatSnap. All rights reserved.Legal|Privacy policy|Modern Slavery Act Transparency Statement|Sitemap