Method for extracting fault feature of antifriction bearing based on sliding entropy-ICA algorithm
A technology of fault characteristics and extraction methods, applied in mechanical bearing testing, mechanical component testing, machine/structural component testing, etc., can solve problems such as inability to accurately predict fault results, complex working environment, and small structures
- Summary
- Abstract
- Description
- Claims
- Application Information
AI Technical Summary
Problems solved by technology
Method used
Image
Examples
Embodiment approach 1
[0080] will be like image 3 The bearing vibration data experiment platform shown in the figure collects single-channel measured signals of four bearing states. The sampling frequency is 20kHz, the bearing model is Rexford ZA-2115, the spindle speed is 2000r / min, and the time domain of the four state signals is Waveform diagram such as Figure 4 As shown, the vibration signals of the four states are decomposed by EMD, and the obtained IMF separation theories are mutually orthogonal and independent, which meets the fact that the actual signals collected by multiple sensors are independent of each other. The steps of EMD decomposing the single-channel measured signal are as follows:
[0081] (1) Split the single-channel measured signal x(t) into the sum of the first-order intrinsic mode function and its residual term, expressed as the following formula:
[0082] x(t)=imf 1 (t)+r 1 (t)
[0083] where r 1 (t) is the residual component of the first-order intrinsic mode functio...
Embodiment approach 2
[0088] According to the n IMF components described in Embodiment 1, the effective IMF components are screened using the sliding entropy correlation coefficient; the sliding entropy correlation coefficient can be used to judge the similarity between two signal components, and the larger the result, the correlation between the two signals The higher the degree; using the sliding entropy correlation coefficient, we can judge the similarity between each IMF component in the EMD decomposition and the original signal component, and then judge the effective virtual channel signal. The calculation steps of the sliding entropy correlation coefficient between the signal x(n) and the IMF component are as follows Shown:
[0089] (1) signal x (n) is divided into k segment length l=20 equally divided length signal segments;
[0090] (2) with x i , i=0,1,2,...,n-l+1 as the starting point, intercept l time series sequentially backwards, use the formula to calculate the entropy value H(i) of ...
Embodiment approach 3
[0099] According to the effective IMF component described in Embodiment 2, it and the single-channel measured signal x(n) form a mixed signal matrix X(t)=[x 1 (t), imf i (t), imf i+1 (t)...], use the FastICA algorithm to perform blind source separation, and obtain the estimated value of the source signal y(t) as Figure 5 As shown, the specific steps of the FastICA algorithm are as follows:
[0100] (2) Preprocessing the observed signal: mainly data centralization and whitening processing; centralization refers to the mean value processing of the signal, that is, subtracting the mean value of the signal sample from each signal sample to obtain:
[0101]
[0102] Signal whitening refers to the linear transformation of the centered observed signal X(t) make The covariance matrix of is a diagonal matrix, so as to remove the correlation between observations; the purpose of centering and whitening is to make the separation matrix W must exist WW T =1, and reduce the param...
PUM
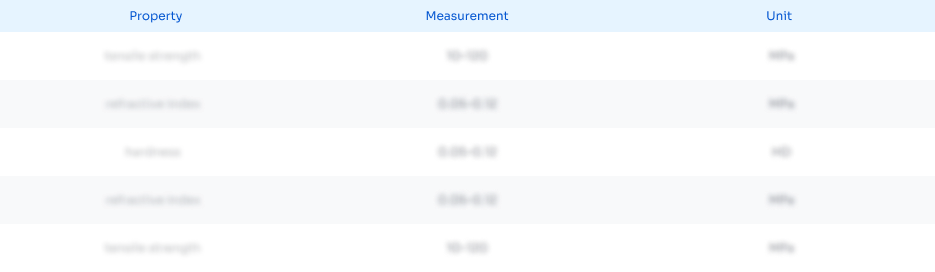
Abstract
Description
Claims
Application Information
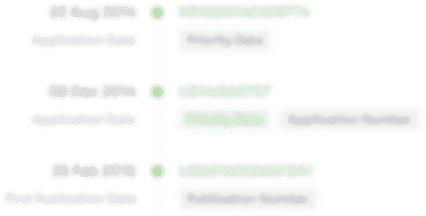
- R&D
- Intellectual Property
- Life Sciences
- Materials
- Tech Scout
- Unparalleled Data Quality
- Higher Quality Content
- 60% Fewer Hallucinations
Browse by: Latest US Patents, China's latest patents, Technical Efficacy Thesaurus, Application Domain, Technology Topic, Popular Technical Reports.
© 2025 PatSnap. All rights reserved.Legal|Privacy policy|Modern Slavery Act Transparency Statement|Sitemap|About US| Contact US: help@patsnap.com