Neural network deep learning-based cloth defect detection method
A deep learning and defect detection technology, applied in the field of fabric defect detection based on neural network deep learning, can solve the problems of high defect similarity, difficult to distinguish, poor production conditions, etc., to achieve the effect of accurate error calculation and improved network robustness
- Summary
- Abstract
- Description
- Claims
- Application Information
AI Technical Summary
Problems solved by technology
Method used
Image
Examples
Embodiment Construction
[0056] The present invention will be further described below in conjunction with specific embodiment:
[0057] See attached Figure 1-6 As shown, the cloth defect detection method based on neural network deep learning described in this embodiment includes the following steps:
[0058] (1) High-speed line scan imaging;
[0059] The high-speed line scan imaging system is built based on the GPU+FPGA structure. The system includes a linear CCD sensor, FPGA, dual-port RAM, GPU, analog-to-digital converter, and multiple storage devices. The imaging steps are as follows:
[0060] 1) FPGA controls the linear CCD sensor to collect image data of cloth;
[0061] 2) The linear CCD sensor transmits the collected cloth image data to the analog-to-digital converter, so that the analog signal is converted into a digital signal, and the converted image signal is stored in the off-chip SDRAM;
[0062] 3) The GPU completes the fast reading operation of the internal image of the dual-port RAM ...
PUM
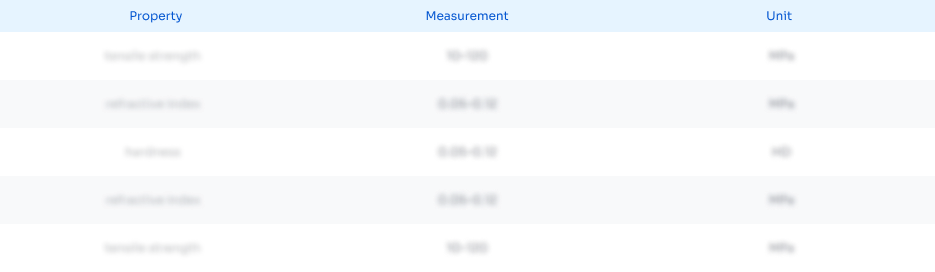
Abstract
Description
Claims
Application Information
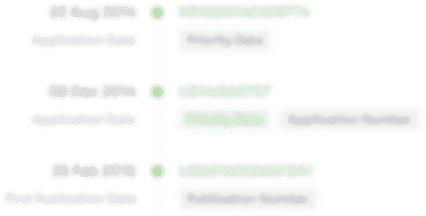
- R&D Engineer
- R&D Manager
- IP Professional
- Industry Leading Data Capabilities
- Powerful AI technology
- Patent DNA Extraction
Browse by: Latest US Patents, China's latest patents, Technical Efficacy Thesaurus, Application Domain, Technology Topic, Popular Technical Reports.
© 2024 PatSnap. All rights reserved.Legal|Privacy policy|Modern Slavery Act Transparency Statement|Sitemap|About US| Contact US: help@patsnap.com