Visual saliency detection method combining machine learning, background suppression and perception positive feedback
A machine learning and background suppression technology, applied in the field of human visual simulation, can solve problems such as information one-way mapping, high hardware resources, manual design, etc.
- Summary
- Abstract
- Description
- Claims
- Application Information
AI Technical Summary
Problems solved by technology
Method used
Image
Examples
Embodiment Construction
[0020] The present invention will be further described below with regard to specific examples, but the present invention is not limited only to these examples.
[0021] The present invention covers any alternatives, modifications, equivalent methods and schemes made on the spirit and scope of the present invention. In order to provide the public with a thorough understanding of the present invention, specific details are set forth in the following preferred embodiments of the present invention, but those skilled in the art can fully understand the present invention without the description of these details. In addition, for the sake of illustration, the drawings of the present invention are not completely drawn according to the actual scale, and are described here.
[0022] The human visual system can detect salient objects and focus attention on regions relevant to the current visual task. Neuropsychological experiments have shown that these abilities are all due to the mecha...
PUM
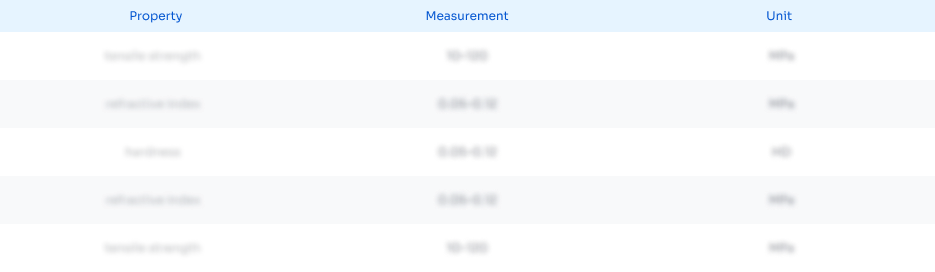
Abstract
Description
Claims
Application Information
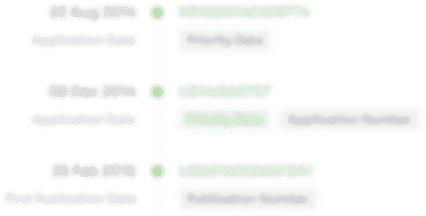
- Generate Ideas
- Intellectual Property
- Life Sciences
- Materials
- Tech Scout
- Unparalleled Data Quality
- Higher Quality Content
- 60% Fewer Hallucinations
Browse by: Latest US Patents, China's latest patents, Technical Efficacy Thesaurus, Application Domain, Technology Topic, Popular Technical Reports.
© 2025 PatSnap. All rights reserved.Legal|Privacy policy|Modern Slavery Act Transparency Statement|Sitemap|About US| Contact US: help@patsnap.com