Multispectral Image Classification Method Based on Recoding and Deep Fusion Convolutional Networks
A multi-spectral image and convolutional network technology, applied in the field of multi-spectral image classification, can solve the problems of high classification accuracy, difficult to achieve, and inability to make full use of data information, and achieve the effect of improving classification accuracy and enhancing generalization ability
- Summary
- Abstract
- Description
- Claims
- Application Information
AI Technical Summary
Problems solved by technology
Method used
Image
Examples
Embodiment Construction
[0047] The present invention will be further described below in conjunction with the accompanying drawings.
[0048] refer to figure 1 , the specific implementation steps of the present invention are as follows:
[0049] Step 1, input the multispectral image to be classified.
[0050] The images to be classified select the multispectral images of five cities obtained by Sentinel-2 satellite. The five cities are: Berlin (Germany), Hong Kong (China), Paris (France), Rome (Italy), and Sao Paulo (Brazil). A given multispectral image is partially labeled. There are 17 categories in total.
[0051] In step 2, the data of different bands in each given city image are stacked together to obtain the 3D image feature FA of the data source.
[0052] Step 3, input the classification auxiliary data of the multispectral image to be classified.
[0053] The classification auxiliary data uses the Osm Raster data corresponding to the five cities in step 1. The Osm Raster data comes from th...
PUM
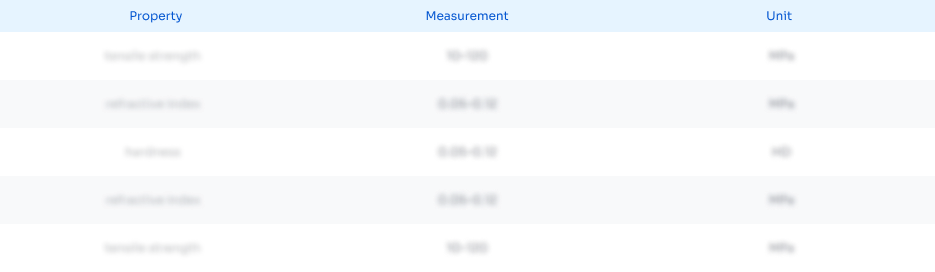
Abstract
Description
Claims
Application Information
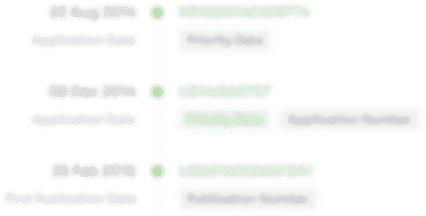
- Generate Ideas
- Intellectual Property
- Life Sciences
- Materials
- Tech Scout
- Unparalleled Data Quality
- Higher Quality Content
- 60% Fewer Hallucinations
Browse by: Latest US Patents, China's latest patents, Technical Efficacy Thesaurus, Application Domain, Technology Topic, Popular Technical Reports.
© 2025 PatSnap. All rights reserved.Legal|Privacy policy|Modern Slavery Act Transparency Statement|Sitemap|About US| Contact US: help@patsnap.com