Remote sensing image ground object classification method based on superpixel coding and convolution neural network
A convolutional neural network and remote sensing image technology, applied in image analysis, image enhancement, image data processing, etc., can solve problems such as low classification accuracy and difficult classification tasks, and achieve the effect of improving classification accuracy and accurate classification results
- Summary
- Abstract
- Description
- Claims
- Application Information
AI Technical Summary
Problems solved by technology
Method used
Image
Examples
Embodiment Construction
[0048] The invention provides a remote sensing image object classification method based on superpixel coding and convolutional neural network, using adaptive superpixel coding and dual-channel convolutional neural network, first using superpixel algorithm to pre-segment images, and then using aggregation The class method merges adjacent and similar superpixel blocks, determines the block size of samples according to the size of the merged pixel blocks, and realizes the adaptive selection of the neighborhood information used. In order to make the classification results more accurate, a dual-channel convolutional neural network is used to extract the features of the two sensor data respectively, and then the extracted features are fused for classification. Set three block sizes, construct three dual-channel convolutional neural networks with different input sizes accordingly, and input samples with different block sizes into the corresponding networks.
[0049] see figure 1 wit...
PUM
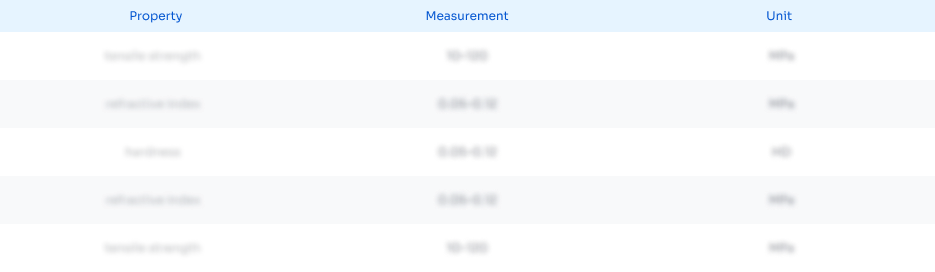
Abstract
Description
Claims
Application Information
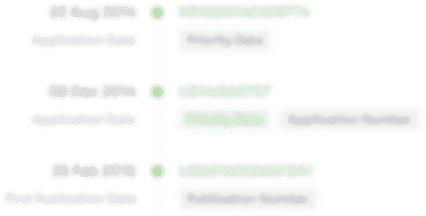
- R&D Engineer
- R&D Manager
- IP Professional
- Industry Leading Data Capabilities
- Powerful AI technology
- Patent DNA Extraction
Browse by: Latest US Patents, China's latest patents, Technical Efficacy Thesaurus, Application Domain, Technology Topic, Popular Technical Reports.
© 2024 PatSnap. All rights reserved.Legal|Privacy policy|Modern Slavery Act Transparency Statement|Sitemap|About US| Contact US: help@patsnap.com