EEMD-ARIMA based cloud computing server load short-term prediction method
A cloud computing server and short-term forecasting technology, applied in computing, computer components, instruments, etc., can solve problems such as server load non-stationary state, difficult to collect sample data, large sample data, etc.
- Summary
- Abstract
- Description
- Claims
- Application Information
AI Technical Summary
Problems solved by technology
Method used
Image
Examples
Embodiment Construction
[0047] The present invention will be further described below in conjunction with the accompanying drawings and embodiments.
[0048] figure 1 The flow chart of the cloud computing server load prediction method of the present invention is provided. In this embodiment, taking the CPU resource load (resource utilization rate) of a certain server on the cloud computing platform as an example, the steps of implementing the short-term prediction method based on EEMD-ARIMA are as follows :
[0049] Step a: For a server on the cloud computing platform, periodically collect the server load for 29 days at a frequency of once per hour, including CPU utilization, memory utilization, and disk utilization.
[0050] Step b: Take the CPU load as the column, extract the CPU utilization of the server for preprocessing, set the data of the first 28 days as the training set, and the data of the 29th day as the verification set. Such as figure 2 As shown, the data after the server CPU load pre...
PUM
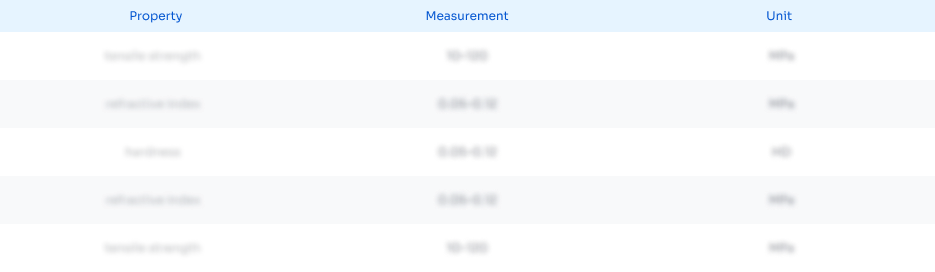
Abstract
Description
Claims
Application Information
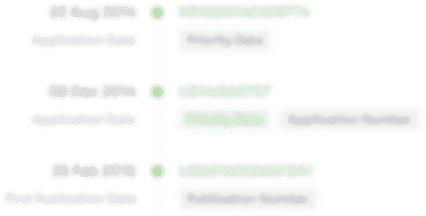
- R&D Engineer
- R&D Manager
- IP Professional
- Industry Leading Data Capabilities
- Powerful AI technology
- Patent DNA Extraction
Browse by: Latest US Patents, China's latest patents, Technical Efficacy Thesaurus, Application Domain, Technology Topic, Popular Technical Reports.
© 2024 PatSnap. All rights reserved.Legal|Privacy policy|Modern Slavery Act Transparency Statement|Sitemap|About US| Contact US: help@patsnap.com