A load balancing access method for Internet of Vehicles based on history reinforcement learning
A technology of reinforcement learning and load balancing, applied in access restriction, network traffic/resource management, electrical components, etc., can solve problems such as network load balancing, and achieve the effect of improving service speed and good network service experience
- Summary
- Abstract
- Description
- Claims
- Application Information
AI Technical Summary
Problems solved by technology
Method used
Image
Examples
Embodiment Construction
[0037] Below in conjunction with specific embodiment, further illustrate the present invention.
[0038] Reinforcement learning focuses on how an agent, the base station, can take a sequence of actions in an environment so as to maximize cumulative rewards. Trial and error and delayed rewards are two distinctive features of reinforcement learning. By continuously interacting with the unknown environment, an agent should know what action to take in what state.
[0039] The load-balancing access method of the Internet of Vehicles based on historical reinforcement learning provided in this embodiment is composed of two parts: the initial reinforcement learning module and the history reinforcement learning module, such as figure 1 shown.
[0040] exist figure 1 In the system architecture described above, firstly, the access base station allocation mode of the vehicle is obtained through the initial reinforcement learning module. These access base station allocation modes are c...
PUM
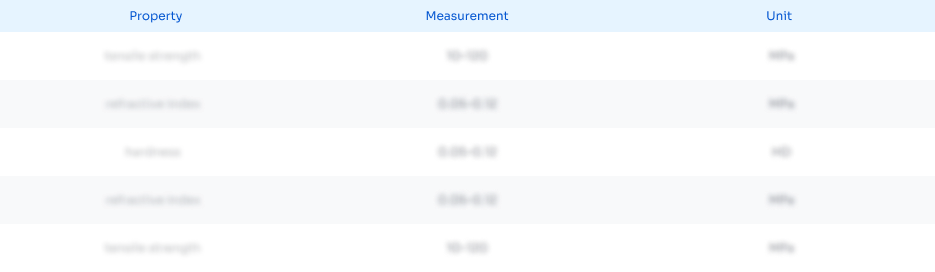
Abstract
Description
Claims
Application Information
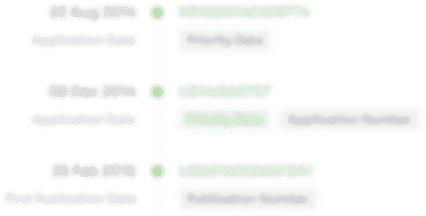
- R&D Engineer
- R&D Manager
- IP Professional
- Industry Leading Data Capabilities
- Powerful AI technology
- Patent DNA Extraction
Browse by: Latest US Patents, China's latest patents, Technical Efficacy Thesaurus, Application Domain, Technology Topic.
© 2024 PatSnap. All rights reserved.Legal|Privacy policy|Modern Slavery Act Transparency Statement|Sitemap