Multiple fading factor-based adaptive target tracking filtering method
A fading factor and target tracking technology, applied in the field of target tracking, can solve the problems of complex adaptive estimation of fading factor and poor target tracking accuracy, and achieve high target tracking accuracy, divergence prevention, and simple calculation
- Summary
- Abstract
- Description
- Claims
- Application Information
AI Technical Summary
Problems solved by technology
Method used
Image
Examples
specific Embodiment approach 1
[0020] Specific implementation mode one: as figure 1 As shown, an adaptive target tracking filtering method based on multiple fading factors provided in this embodiment is specifically carried out according to the following steps:
[0021] Step 1, establish the state model and measurement model of the moving target, mark the motion state of the state model as an N-dimensional motion state vector X, and mark the measurement value of the measurement model as an M-dimensional measurement vector Z, according to the motion model Obtain the state error covariance matrix P and the process noise covariance matrix Q, and obtain the measurement noise covariance matrix R according to the measurement model;
[0022] Step 2: Obtain the motion state vector X(k) and the state error covariance matrix P(k) at the kth moment, and calculate the predicted value of the motion state vector of the moving target at the k+1 moment according to the Kalman recursion formula Measure Vector Prediction ...
specific Embodiment approach 2
[0026] Embodiment 2: The difference between this embodiment and Embodiment 1 is that the motion model of the target in step 1 is:
[0027] X(k+1)=F(k)X(k)+G(k)V(k)
[0028] Among them, F(k) is the N×N order state transition matrix, G(k) is the N×M dimensional process noise distribution matrix, V(k) is the M dimensional process noise, which is Gaussian white noise with zero mean, The process noise covariance matrix is Q(k).
specific Embodiment approach 3
[0029] Specific implementation mode three: the difference between this implementation mode and specific implementation mode two is: the measurement model of the target in step one is:
[0030] Z(k)=H(k)X(k)+W(k)
[0031] Among them, H(k) is the measurement matrix of order M×N, W(k) is the measurement noise of M dimension, which is Gaussian white noise with zero mean, and the measurement noise covariance matrix is R(k).
PUM
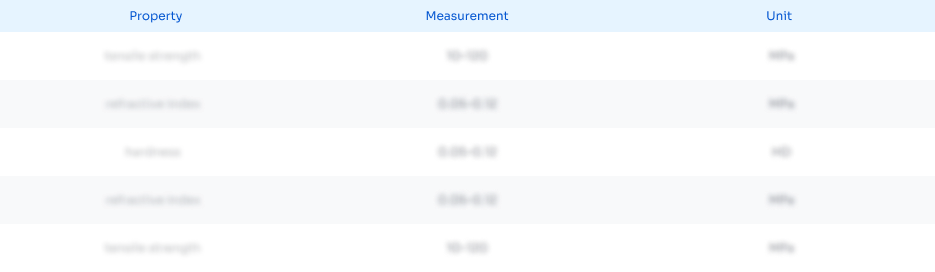
Abstract
Description
Claims
Application Information
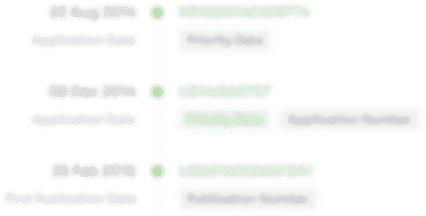
- R&D Engineer
- R&D Manager
- IP Professional
- Industry Leading Data Capabilities
- Powerful AI technology
- Patent DNA Extraction
Browse by: Latest US Patents, China's latest patents, Technical Efficacy Thesaurus, Application Domain, Technology Topic, Popular Technical Reports.
© 2024 PatSnap. All rights reserved.Legal|Privacy policy|Modern Slavery Act Transparency Statement|Sitemap|About US| Contact US: help@patsnap.com