Multi-wave matching method based on convolutional neural network
A convolutional neural network and matching method technology, applied in neural learning methods, biological neural network models, seismic signal processing, etc., can solve the problems of low accuracy, rough accuracy, and no significant progress in geological applications of full-wave attributes. , to achieve the effect of improving matching accuracy and efficiency and reducing workload
- Summary
- Abstract
- Description
- Claims
- Application Information
AI Technical Summary
Problems solved by technology
Method used
Image
Examples
Embodiment Construction
[0036] In order to make the object, technical solution and advantages of the present invention clearer, the present invention will be further described in detail below in conjunction with the accompanying drawings and embodiments. It should be understood that the specific embodiments described here are only used to explain the present invention, not to limit the present invention.
[0037] Such as figure 1 Shown is a schematic flow chart of the convolutional neural network-based multi-wave matching method of the present invention. A multi-wave matching method based on convolutional neural network, comprising the following steps:
[0038] A. Preprocessing the shear wave and longitudinal wave data;
[0039] B. Divide the preprocessed shear wave and longitudinal wave data into spatial grids according to the preset step size in step A;
[0040] C, calculating the grid point displacement of the spatial grid in step B;
[0041] D. Fusion of shear wave and longitudinal wave data ...
PUM
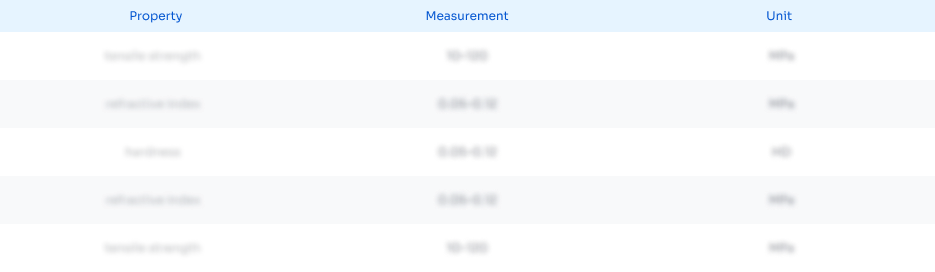
Abstract
Description
Claims
Application Information
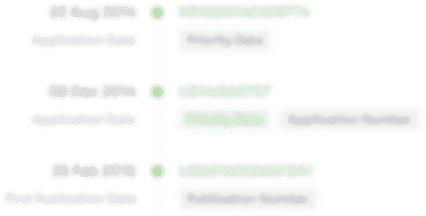
- R&D
- Intellectual Property
- Life Sciences
- Materials
- Tech Scout
- Unparalleled Data Quality
- Higher Quality Content
- 60% Fewer Hallucinations
Browse by: Latest US Patents, China's latest patents, Technical Efficacy Thesaurus, Application Domain, Technology Topic, Popular Technical Reports.
© 2025 PatSnap. All rights reserved.Legal|Privacy policy|Modern Slavery Act Transparency Statement|Sitemap|About US| Contact US: help@patsnap.com