A Recognition Method Based on Hand-drawn Sketch
A recognition method and sketch technology, applied in character and pattern recognition, still image data retrieval, metadata still image retrieval, etc., can solve the problem of inability to realize the semantic understanding of sketches, and achieve accurate and fast retrieval results, multiple database categories, and retrieval. Precise results
- Summary
- Abstract
- Description
- Claims
- Application Information
AI Technical Summary
Problems solved by technology
Method used
Image
Examples
Embodiment 1
[0033] A recognition method based on hand-drawn sketches, see figure 1 , the method includes the following steps:
[0034] 101: Adjust each sketch in the initial category set according to the preset size, uniformly extract several interest points, and extract a square block for each interest point;
[0035] 102: Extract pixel point gradients in each square block, and quantify to units in 4 directions according to the direction, as the local features of each square block; use k-means clustering method to construct a visual dictionary, and each sketch uses 500-dimensional vector representation;
[0036] 103: Using intra-class clustering analysis and performing dimensionality reduction processing on the feature vectors of each category to obtain the classified database;
[0037] 104: Match the query sketch with the classified database to obtain the final retrieval result.
[0038] Wherein, the initial category set in step 101 is specifically:
[0039] A total of 20,000 sketch...
Embodiment 2
[0046] The scheme in embodiment 1 is further introduced below in conjunction with specific calculation formulas and examples, see the following description for details:
[0047] 201: Obtain a total of 20,000 sketch datasets, make keywords cover most common categories through preprocessing, and use the preprocessed sketch dataset as the initial category set;
[0048] Wherein, the step 201 specifically includes:
[0049] 1) First construct a sketch data set of 20,000 pieces as the basis for learning, evaluation and application;
[0050] 2) Extract 1000 most common labels from LabelMe (label library), and manually remove duplicates and non-compliance rules with 1000 common labels as a benchmark (set according to the needs in practical applications, the embodiment of the present invention There is no limit to this, e.g. labels for chairs, mugs);
[0051] 3) Supplement the deleted draft dataset with keywords through preset standards and keywords in the preset dataset, and use the...
Embodiment 3
[0101] Below in conjunction with concrete experimental data, mathematical formula, the scheme in embodiment 1 and 2 is carried out feasibility verification, see the following description for details:
[0102] experimental report
[0103] 1. Database
[0104] A collection of 20,000 hand-drawn sketches databases that are the basis for learning, evaluation and application. Extract 1000 most common labels from LabelMe, manually remove duplicate and irregular labels, and use this as the initial category set. Increase the number of categories by keywords from PSB and Caltech 256 datasets. Finally, keywords are artificially supplemented, and finally 250 keywords are obtained, and these words cover most of the common categories.
[0105] Using the full 250 classes, each sketch was converted to a grayscale bitmap and sized to 256×256. 28×28=784 local features are extracted for each sketch, and one feature is extracted in each grid. In order to create a visual dictionary, a huge numb...
PUM
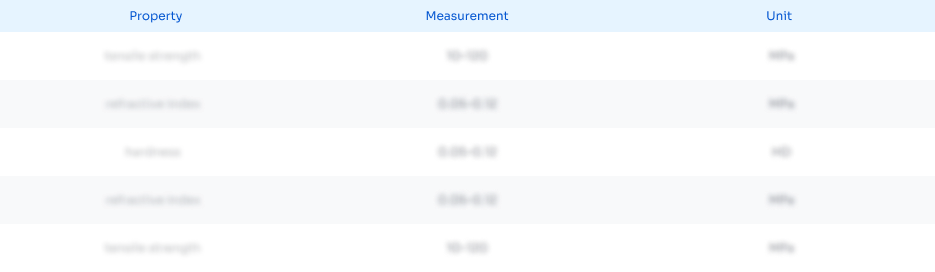
Abstract
Description
Claims
Application Information
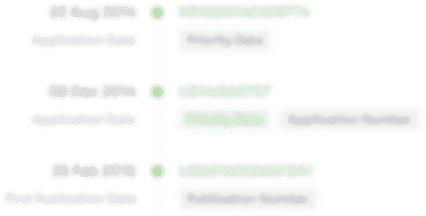
- R&D
- Intellectual Property
- Life Sciences
- Materials
- Tech Scout
- Unparalleled Data Quality
- Higher Quality Content
- 60% Fewer Hallucinations
Browse by: Latest US Patents, China's latest patents, Technical Efficacy Thesaurus, Application Domain, Technology Topic, Popular Technical Reports.
© 2025 PatSnap. All rights reserved.Legal|Privacy policy|Modern Slavery Act Transparency Statement|Sitemap|About US| Contact US: help@patsnap.com