Risk identification method and system based on transfer deep learning
A technology of risk identification and deep learning, applied in the field of transaction risk identification method and system based on migration deep learning, can solve the problem of difficult identification of unknown fraud types, and achieve the effect of accurate identification
- Summary
- Abstract
- Description
- Claims
- Application Information
AI Technical Summary
Problems solved by technology
Method used
Image
Examples
Embodiment Construction
[0043] Introduced below are some of the various embodiments of the invention, intended to provide a basic understanding of the invention. It is not intended to identify key or critical elements of the invention or to delineate the scope of protection.
[0044] First, several concepts to be mentioned in the present invention are described.
[0045] (1) Restricted Boltzmann Machine (RBM)
[0046] RBM is a stochastically generated neural network that learns a probability distribution over an input dataset. RBM is a variant of Boltzmann machine, but the limited model must be a bipartite graph. The model contains visible units corresponding to input parameters (hereinafter also referred to as visible layers) and hidden units corresponding to training results (hereinafter also referred to as hidden layers), and each edge must connect a visible unit and a hidden unit.
[0047] (2) BP algorithm (that is, error backpropagation algorithm)
[0048] The BP algorithm is a learning algori...
PUM
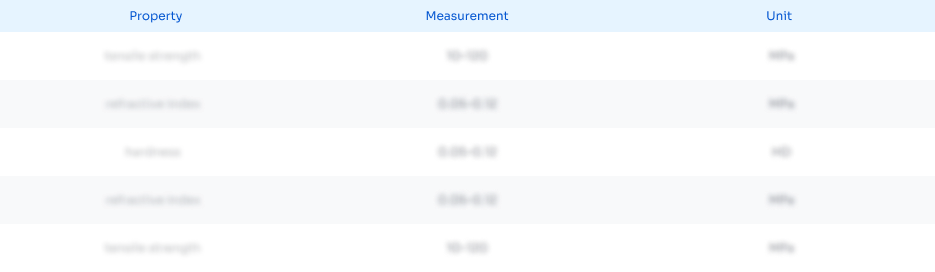
Abstract
Description
Claims
Application Information
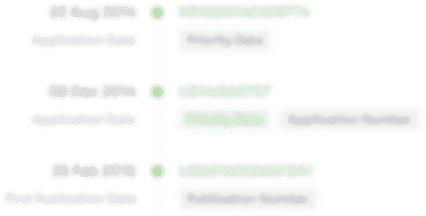
- R&D
- Intellectual Property
- Life Sciences
- Materials
- Tech Scout
- Unparalleled Data Quality
- Higher Quality Content
- 60% Fewer Hallucinations
Browse by: Latest US Patents, China's latest patents, Technical Efficacy Thesaurus, Application Domain, Technology Topic, Popular Technical Reports.
© 2025 PatSnap. All rights reserved.Legal|Privacy policy|Modern Slavery Act Transparency Statement|Sitemap|About US| Contact US: help@patsnap.com