Fabric defect detection method based on deep convolution neural network and visual saliency
A neural network and deep convolution technology, applied in the field of visual inspection, can solve problems such as large impact and poor defect detection effect, achieve strong real-time performance, meet actual engineering needs, and have wide application prospects
- Summary
- Abstract
- Description
- Claims
- Application Information
AI Technical Summary
Problems solved by technology
Method used
Image
Examples
Embodiment Construction
[0020] The specific implementation manners of the present invention will be further described in detail below in conjunction with the accompanying drawings.
[0021] Such as figure 1 and figure 2 As shown, the fabric defect detection method based on the deep convolutional neural network and visual salience of the present invention includes a defect region localization network model and a defect segmentation network model. The defect location network model uses the fusion of the global neural network model and the local neural network model to provide accurate location information of defects in the fabric image. The defect segmentation network model uses superpixels and visual saliency content to segment defect regions and extract defect targets. Include the following steps:
[0022] (1) Select the fabric defect training data set, carry out grayscale processing to the images in the data set, and then carry out size normalization processing;
[0023] (2) Input the fabric de...
PUM
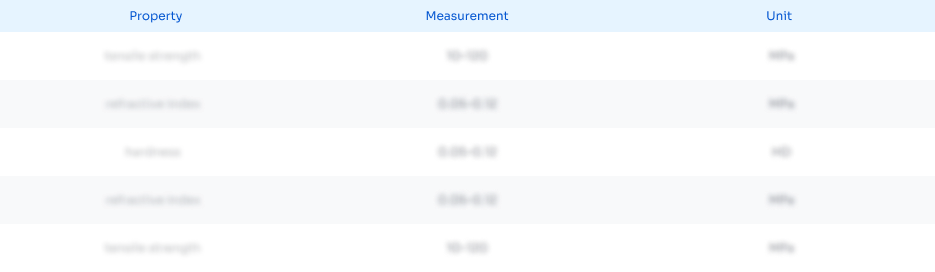
Abstract
Description
Claims
Application Information
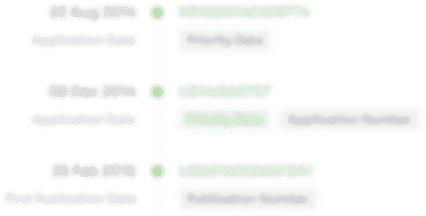
- R&D
- Intellectual Property
- Life Sciences
- Materials
- Tech Scout
- Unparalleled Data Quality
- Higher Quality Content
- 60% Fewer Hallucinations
Browse by: Latest US Patents, China's latest patents, Technical Efficacy Thesaurus, Application Domain, Technology Topic, Popular Technical Reports.
© 2025 PatSnap. All rights reserved.Legal|Privacy policy|Modern Slavery Act Transparency Statement|Sitemap|About US| Contact US: help@patsnap.com