Fine granularity vehicle multi-property recognition method based on convolutional neural network
A convolutional neural network and neural network technology, applied in the field of fine-grained vehicle multi-attribute recognition based on convolutional neural network, can solve practical problems that have not been discussed, and achieve the effect of reducing human intervention
- Summary
- Abstract
- Description
- Claims
- Application Information
AI Technical Summary
Problems solved by technology
Method used
Image
Examples
Embodiment Construction
[0049] The preferred embodiments of the present invention will be described in detail below with reference to the accompanying drawings.
[0050] Such as figure 1 as shown, figure 1It is a schematic diagram of the method for realizing the present invention. It includes two modules: training module and testing module. The training module is the training process of the neural network. The training data set and label data are passed through the multi-task neural network to obtain the neural model. The testing module is the neural network testing process. The test data set undergoes the feature extraction process and the final output is obtained through the trained neural model.
[0051] Such as figure 2 as shown, figure 2 for L softmax and L triplet The joint learning network structure model of , the steps are as follows:
[0052] Step 201: Obtain a fine-grained vehicle dataset, and divide vehicle images into three-tuple models which is reference sample, yes and ...
PUM
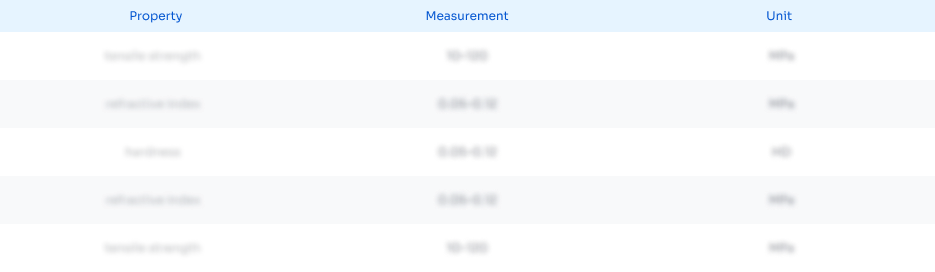
Abstract
Description
Claims
Application Information
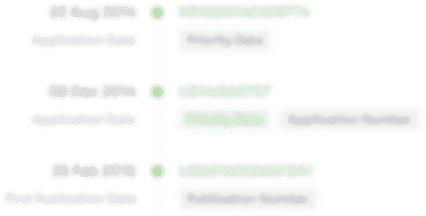
- R&D Engineer
- R&D Manager
- IP Professional
- Industry Leading Data Capabilities
- Powerful AI technology
- Patent DNA Extraction
Browse by: Latest US Patents, China's latest patents, Technical Efficacy Thesaurus, Application Domain, Technology Topic, Popular Technical Reports.
© 2024 PatSnap. All rights reserved.Legal|Privacy policy|Modern Slavery Act Transparency Statement|Sitemap|About US| Contact US: help@patsnap.com