Real-time dense monocular SLAM method and system based on online learning depth prediction network
A depth prediction and dense technology, applied in biological neural network models, image analysis, instruments, etc., can solve the problems of lack of scale information and inability to realize dense mapping in monocular SLAM, and achieve the effect of improving accuracy and accuracy
- Summary
- Abstract
- Description
- Claims
- Application Information
AI Technical Summary
Problems solved by technology
Method used
Image
Examples
Embodiment Construction
[0045] In order to make the object, technical solution and advantages of the present invention clearer, the present invention will be further described in detail below in conjunction with the accompanying drawings and embodiments. It should be understood that the specific embodiments described here are only used to explain the present invention, not to limit the present invention. In addition, the technical features involved in the various embodiments of the present invention described below can be combined with each other as long as they do not constitute a conflict with each other.
[0046] The problem to be solved by the present invention is to realize a real-time monocular dense mapping SLAM system. The system adopts the combination of self-adaptive online CNN depth prediction network and monocular SLAM system based on direct method, which can not only significantly improve the performance of unknown scene The accuracy and robustness of depth prediction can also solve the ...
PUM
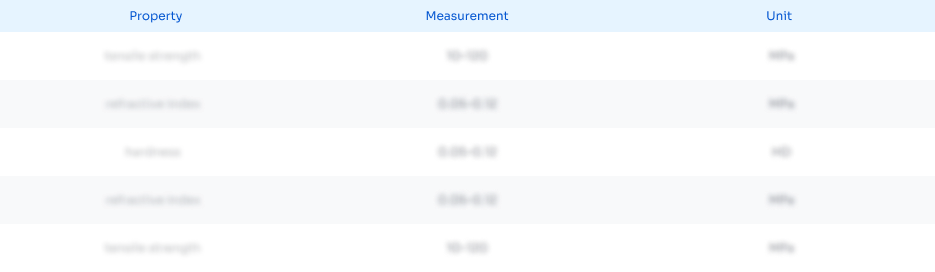
Abstract
Description
Claims
Application Information
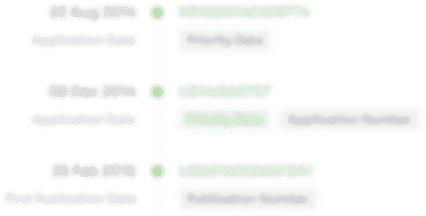
- R&D Engineer
- R&D Manager
- IP Professional
- Industry Leading Data Capabilities
- Powerful AI technology
- Patent DNA Extraction
Browse by: Latest US Patents, China's latest patents, Technical Efficacy Thesaurus, Application Domain, Technology Topic, Popular Technical Reports.
© 2024 PatSnap. All rights reserved.Legal|Privacy policy|Modern Slavery Act Transparency Statement|Sitemap|About US| Contact US: help@patsnap.com