Dynamic model identification method and system based on recurrent neural network
A cyclic neural network and dynamic model technology, applied in general control systems, control/regulation systems, instruments, etc., can solve the problem of increasing the chance of errors, less able to obtain the transfer function model more accurately, difficult to find the results and find them. problems, etc.
- Summary
- Abstract
- Description
- Claims
- Application Information
AI Technical Summary
Problems solved by technology
Method used
Image
Examples
Embodiment
[0174] Example: A 1000MW supercritical unit leading air temperature model is at three load points of 1000MW, 750MW, and 500MW, and the step characteristic test of the opening of the flue gas baffle on the leading air temperature is carried out, and the relevant mathematical parameters are obtained through model fitting. Model.
[0175]
[0176] Setting the sampling period to 1s, the following discrete-time model can be obtained:
[0177] y'(k)=[480y'(k-1)+u'(k)-225y'(k-2)] / 256 (25);
[0178] In the formula, y'(k) represents the output of the model at the current moment; u'(k) represents the input of the model at the current moment.
[0179] On the basis of this model, it is assumed that the nonlinear model is:
[0180] y”(k)=[480y”(k-1)+u”(k) 2 -225y”(k-2)] / 256 (26);
[0181] In the formula, y"(k) represents the output of the model at the current moment; u"(k) represents the input of the model at the current moment.
[0182] The linear kernel function is used, and N is...
PUM
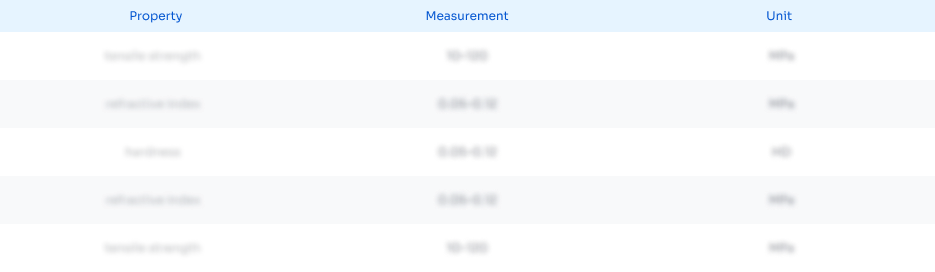
Abstract
Description
Claims
Application Information
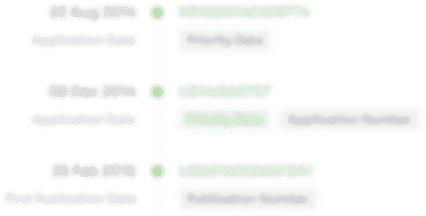
- R&D
- Intellectual Property
- Life Sciences
- Materials
- Tech Scout
- Unparalleled Data Quality
- Higher Quality Content
- 60% Fewer Hallucinations
Browse by: Latest US Patents, China's latest patents, Technical Efficacy Thesaurus, Application Domain, Technology Topic, Popular Technical Reports.
© 2025 PatSnap. All rights reserved.Legal|Privacy policy|Modern Slavery Act Transparency Statement|Sitemap|About US| Contact US: help@patsnap.com