A Face Age Estimation Method Based on Convolutional Neural Network for Distribution Learning
A convolutional neural network and network technology, applied in neural learning methods, biological neural network models, calculations, etc., can solve problems such as difficulty including, ignoring age-ordered age correlation, and unsatisfactory age distribution, so as to achieve accurate age estimation , the effect of less manual intervention
- Summary
- Abstract
- Description
- Claims
- Application Information
AI Technical Summary
Problems solved by technology
Method used
Image
Examples
Embodiment Construction
[0024] The present invention will be described in further detail below with reference to the accompanying drawings and specific embodiments.
[0025] A human face age estimation method based on convolutional neural networks, the overall steps are:
[0026] Step 1, the data extraction phase: Using the existing face detection engine to face the face RGB image to face the face RGB image, 5 points are positioned in two corners, nose, two mouths; then cut people The face area saves the face image to 256 × 256 pixels.
[0027] Step II. Age data set division: In order to ensure the generalization of the model, avoid being equipped on the training set, need to be divided on the data set; the age data set is taken as a training set, 20% as a verification set is randomly Divide, to ensure that the same person's data appears only in a collection. Age data set includes a face image of different age categories.
[0028] Step 3, age estimation network structure: Age estimation network model ado...
PUM
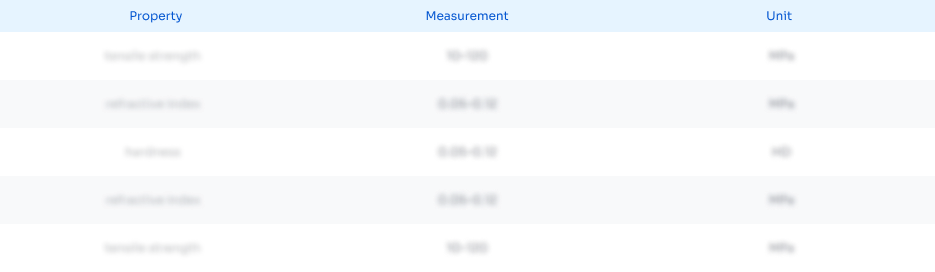
Abstract
Description
Claims
Application Information
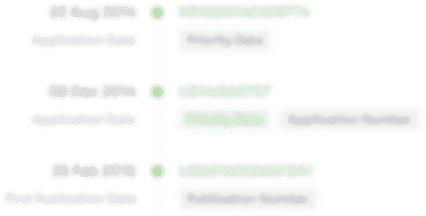
- R&D Engineer
- R&D Manager
- IP Professional
- Industry Leading Data Capabilities
- Powerful AI technology
- Patent DNA Extraction
Browse by: Latest US Patents, China's latest patents, Technical Efficacy Thesaurus, Application Domain, Technology Topic, Popular Technical Reports.
© 2024 PatSnap. All rights reserved.Legal|Privacy policy|Modern Slavery Act Transparency Statement|Sitemap|About US| Contact US: help@patsnap.com