Quantitative photoacoustic imaging method on basis of deep neural networks
A technology of deep neural network and photoacoustic imaging, which is applied in the field of quantitative photoacoustic imaging based on deep neural network, can solve the problems of error, large-scale high-resolution image reconstruction and large amount of calculation, and achieve high accuracy, fast image reconstruction, easily optimized effects
- Summary
- Abstract
- Description
- Claims
- Application Information
AI Technical Summary
Problems solved by technology
Method used
Image
Examples
Embodiment Construction
[0012] The present invention will be described in detail below in conjunction with the accompanying drawings. However, it should be understood that the accompanying drawings are provided only for better understanding of the present invention, and they should not be construed as limiting the present invention.
[0013] Deep learning (DL) has attracted attention in many fields, including medical imaging. Using a special deep neural network (DNN) to represent nonlinear mappings, and adjusting network weights through large training data, DL can automatically detect features from measurement data, and use the mined features to predict target data or perform decision making.
[0014] Convolutional neural network (CNN) has superior image processing performance, and the neural layer of CNN can filter input data and extract useful information. U-net is a fully convolutional neural network. U-net consists of a contraction path (to capture environmental information) and a symmetrical ...
PUM
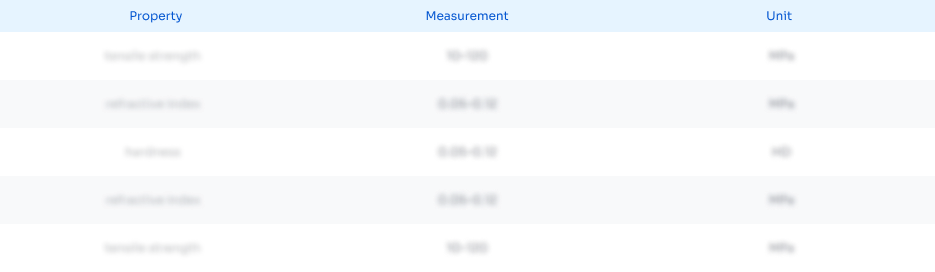
Abstract
Description
Claims
Application Information
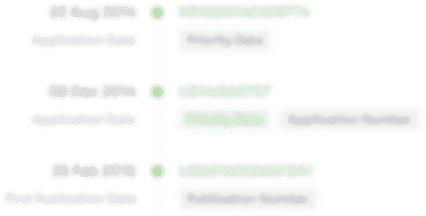
- R&D
- Intellectual Property
- Life Sciences
- Materials
- Tech Scout
- Unparalleled Data Quality
- Higher Quality Content
- 60% Fewer Hallucinations
Browse by: Latest US Patents, China's latest patents, Technical Efficacy Thesaurus, Application Domain, Technology Topic, Popular Technical Reports.
© 2025 PatSnap. All rights reserved.Legal|Privacy policy|Modern Slavery Act Transparency Statement|Sitemap|About US| Contact US: help@patsnap.com