3D Model Segmentation Method Based on Fusion of Multi-view Features by Projective Fully Convolutional Network
A fully convolutional network and multi-view technology, applied in the field of projected full convolutional network 3D model segmentation, can solve problems such as low practicality, prolonging the training time of the framework, and time-consuming viewing angle selection
- Summary
- Abstract
- Description
- Claims
- Application Information
AI Technical Summary
Problems solved by technology
Method used
Image
Examples
Embodiment
[0104] The object task of the present invention is as Figure 1a and Figure 1b as shown, Figure 1a is the undivided original model, Figure 1b Coloring and rendering results of labels after semantic segmentation, the structure of the whole method is as follows figure 2 shown. Each step of the present invention is described below according to an embodiment.
[0105] Step (1), collect data on the input 3D grid model data set S. Taking model s as an example, it is divided into the following steps:
[0106] In step (1.1), select 14 viewpoints from 42 fixed viewpoints to maximize the model patch coverage;
[0107] Step (1.1.1), set 42 fixed viewpoints, such as image 3 As shown, the distance from the viewpoint to the coordinate origin depends on whether the projection images in all viewpoint directions of the model can fill the rendering window as much as possible. In this paper, the size of the experimental rendering window is set to 320×320, and the unit is pixel. There ...
PUM
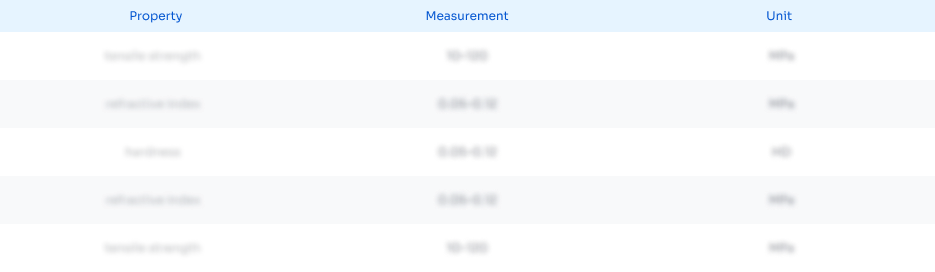
Abstract
Description
Claims
Application Information
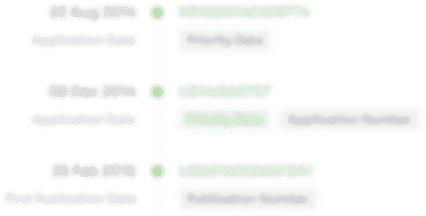
- R&D
- Intellectual Property
- Life Sciences
- Materials
- Tech Scout
- Unparalleled Data Quality
- Higher Quality Content
- 60% Fewer Hallucinations
Browse by: Latest US Patents, China's latest patents, Technical Efficacy Thesaurus, Application Domain, Technology Topic, Popular Technical Reports.
© 2025 PatSnap. All rights reserved.Legal|Privacy policy|Modern Slavery Act Transparency Statement|Sitemap|About US| Contact US: help@patsnap.com