Image classification method fusing radiomics and deep convolution features
A deep convolution and deep feature technology, applied in the field of medical image processing, can solve the problems of reducing the accuracy of medical image classification and making decision difficult.
- Summary
- Abstract
- Description
- Claims
- Application Information
AI Technical Summary
Problems solved by technology
Method used
Image
Examples
Embodiment 1
[0030] A method for image classification by fusing radiomics and deep convolutional features, such as figure 1 shown, including the following steps:
[0031] Step 1, read the image and perform three-dimensional segmentation on the image area to obtain a three-dimensional segmented image;
[0032] Step 2, normalizing the pixels of the three-dimensional segmented image in step 1 to obtain a normalized image;
[0033] Step 3, performing radiomics feature extraction on the normalized image preprocessing of step 2;
[0034] Step 4, screening the radiomics features of step 3 to obtain the radiomics features to be combined;
[0035] Step 5, randomly extracting three-dimensional image blocks with equal probability from the normalized image in step 2;
[0036] Step 6, train the 3D image block in step 5, input the 3D image block into the convolutional neural network for training, and obtain the final depth feature;
[0037] In step seven, the radiomics features in step four and the ...
Embodiment 2
[0050] A method for image classification by fusing radiomics and deep convolution features, combining a data set composed of CT images to describe the processing process of the method of the present invention in detail, the specific steps are as follows:
[0051] Step 1: Read the CT image and import the CT image into the image control software of ITK-SNAP3.xTeam to outline the CT image area and perform three-dimensional segmentation;
[0052] Step 2, by adjusting the window width and window level of the divided CT image to be the default value stored in the DCM file, then using the maximum and minimum algorithm to normalize the pixels of the adjusted image;
[0053] Step 3: Preprocessing the normalized images, mainly including wavelet bandpass filtering, isotropic sampling and grayscale quantization, and then extracting 3D radiomics features, including 10320-dimensional texture features and 4-dimensional non-texture features.
[0054] Step 4, use the feature selection operator R...
PUM
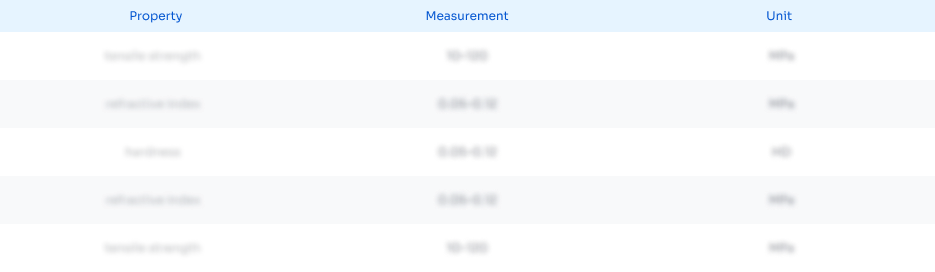
Abstract
Description
Claims
Application Information
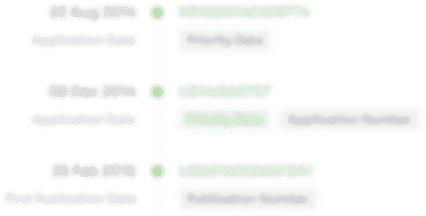
- Generate Ideas
- Intellectual Property
- Life Sciences
- Materials
- Tech Scout
- Unparalleled Data Quality
- Higher Quality Content
- 60% Fewer Hallucinations
Browse by: Latest US Patents, China's latest patents, Technical Efficacy Thesaurus, Application Domain, Technology Topic, Popular Technical Reports.
© 2025 PatSnap. All rights reserved.Legal|Privacy policy|Modern Slavery Act Transparency Statement|Sitemap|About US| Contact US: help@patsnap.com