An Unsupervised Clustering Method for Classification of Large-scale Spectral Remote Sensing Images
A technology of remote sensing image and clustering method, which is applied in the field of spectral remote sensing image ground object classification, to achieve the effects of accelerating classification speed, high computing efficiency, and reducing computing redundancy
- Summary
- Abstract
- Description
- Claims
- Application Information
AI Technical Summary
Problems solved by technology
Method used
Image
Examples
Embodiment Construction
[0045] The present invention will be further described below in conjunction with the accompanying drawings and specific embodiments.
[0046] Technical scheme block diagram of the present invention is as figure 2 As shown, the basic technical principles are as follows.
[0047] Step 1: Spectrum selection.
[0048] Hyperspectral remote sensing images contain hundreds of continuous spectral segments and have a large amount of data. In the process of image processing, the spectral segment selection method is often used to select the optimal feature spectral segment, sacrificing some classification accuracy to greatly improve the efficiency of classification processing. The complexity of various spectral band selection methods is different. Here is a brief introduction to the principal component analysis method in [1].
[0049] As shown in formula (1), assuming that the original image data Y contains N pixels and L spectral segments, the correlation matrix defining the data is...
PUM
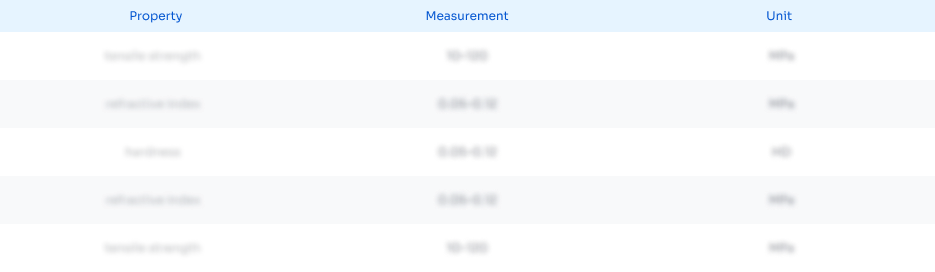
Abstract
Description
Claims
Application Information
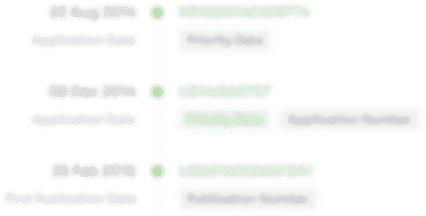
- R&D Engineer
- R&D Manager
- IP Professional
- Industry Leading Data Capabilities
- Powerful AI technology
- Patent DNA Extraction
Browse by: Latest US Patents, China's latest patents, Technical Efficacy Thesaurus, Application Domain, Technology Topic, Popular Technical Reports.
© 2024 PatSnap. All rights reserved.Legal|Privacy policy|Modern Slavery Act Transparency Statement|Sitemap|About US| Contact US: help@patsnap.com