Method for reconstructing sparse MRI (Magnetic resonance imaging) based on convolutional neural network in combination with iterative method
A convolutional neural network and neural network technology, applied in the field of sparse MRI reconstruction, can solve the problems of slow imaging, achieve fast reconstruction speed, retain structure and information, and improve the effect of easy loss of details
- Summary
- Abstract
- Description
- Claims
- Application Information
AI Technical Summary
Problems solved by technology
Method used
Image
Examples
Embodiment Construction
[0033] This embodiment provides a method for sparse MRI reconstruction based on the combination of convolutional neural network and iterative method, comprising the following steps:
[0034] (1) Obtain multiple MRI data sets, transform them into fully sampled k-space data, and then generate down-sampled k-space data through sampling.
[0035] For example, 250 pieces of MRI cardiac data from clinical use in a hospital can be obtained, and Fourier transformation can be performed on the 250 pieces of data to simulate fully sampled k-space data. Then, the radial sampling matrix with a sampling rate of 10% is used to down-sample the fully sampled k-space data to obtain the down-sampled k-space data.
[0036] (2) In the same way, the downsampled k-space data and the full-sampled k-space data are divided into low-frequency data and high-frequency data, and converted to the image domain to obtain down-sampled low-frequency image domain data and down-sampled high-frequency image domain...
PUM
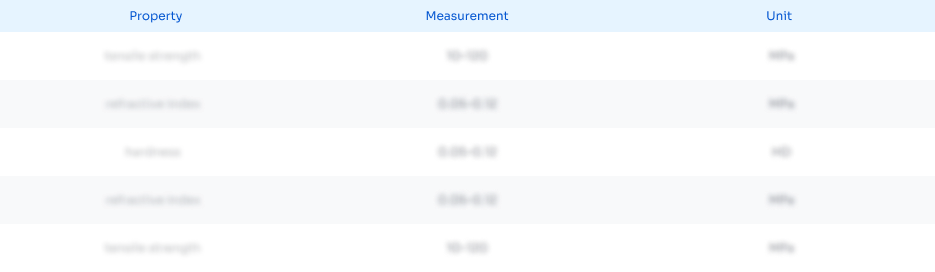
Abstract
Description
Claims
Application Information
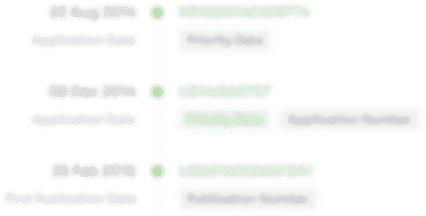
- R&D
- Intellectual Property
- Life Sciences
- Materials
- Tech Scout
- Unparalleled Data Quality
- Higher Quality Content
- 60% Fewer Hallucinations
Browse by: Latest US Patents, China's latest patents, Technical Efficacy Thesaurus, Application Domain, Technology Topic, Popular Technical Reports.
© 2025 PatSnap. All rights reserved.Legal|Privacy policy|Modern Slavery Act Transparency Statement|Sitemap|About US| Contact US: help@patsnap.com