Wind energy prediction method which combines spatial-temporal features and error processing
A spatio-temporal feature and error processing technology, applied in feature engineering, wind energy forecasting, and data mining, can solve problems that are not universal and applicable to specific scenarios, and achieve better consistency, improved prediction accuracy, and strong stability Effect
- Summary
- Abstract
- Description
- Claims
- Application Information
AI Technical Summary
Problems solved by technology
Method used
Image
Examples
Embodiment 1
[0035] In order to achieve the above purpose, the embodiment of the present invention proposes a wind energy prediction method that combines spatio-temporal features and error processing, see figure 1 , the method consists of the following steps:
[0036] 101: Extract time features from wind energy time series, extract information between nearby wind turbines through multi-input-single-output mode, and introduce spatial features;
[0037] On the one hand, the wind energy time series reflects the change of the output electric power of the wind turbine with time, so the characteristic data are extracted from the wind energy time series for training the prediction model.
[0038] On the other hand, in the embodiment of the present invention, wind turbines that are relatively close to each other are referred to as "neighbors". Extract the information between wind turbines at a short distance and introduce spatial features.
[0039] The embodiment of the present invention adopts ...
Embodiment 2
[0057] The following is combined with specific calculation formulas, examples, and figure 2 The scheme in Example 1 is further introduced, see the following description for details:
[0058] 201: During the training process of the VFMLEs model, the data set must first be Group by feature variance;
[0059] 202: Extract spatio-temporal features in corresponding groups. The embodiment of the present invention adopts a multi-input-single-output mode, that is, , where X is a vector, namely a spatio-temporal feature, and y is an output. Spatio-temporal feature extraction methods such as figure 2 shown.
[0060] Such as figure 2 As shown, for a single observation object nt i , the time feature is obtained from the data of a single wind turbine, and the tb sequence is obtained. At any time, several recent measured values in the past are selected as features, and the measured values at a specific time distance in the future are used as the output corresponding to the time...
Embodiment 3
[0086] Below in conjunction with concrete experimental data, the scheme in embodiment 1 and 2 is carried out feasibility verification, see the following description for details:
[0087] The problem of wind power prediction is essentially a numerical prediction problem, and there are general standards for evaluating numerical prediction problems, such as: mean absolute error MAE, mean square error MSE and root mean square error RMSE, etc. Usually, the model is judged by the "error rate (the percentage of the error and the actual value)". This method has certain defects. For example, the value of the error rate depends on the actual value. When the actual value is small, even if the prediction error is small, the error The error rate can also be large, and conversely, when the actual value is large, the error rate can be small even if the model performs poorly. The embodiment of the present invention mainly uses MSE to evaluate and compare the experimental results. The calcula...
PUM
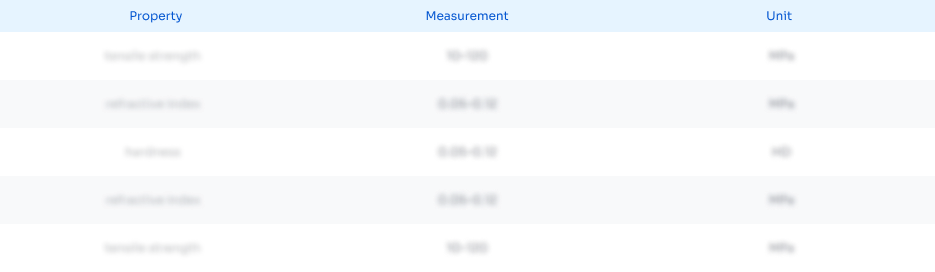
Abstract
Description
Claims
Application Information
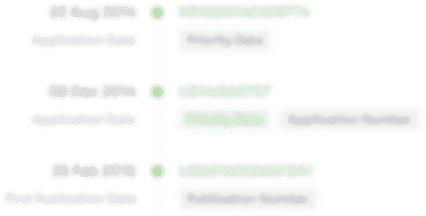
- R&D Engineer
- R&D Manager
- IP Professional
- Industry Leading Data Capabilities
- Powerful AI technology
- Patent DNA Extraction
Browse by: Latest US Patents, China's latest patents, Technical Efficacy Thesaurus, Application Domain, Technology Topic, Popular Technical Reports.
© 2024 PatSnap. All rights reserved.Legal|Privacy policy|Modern Slavery Act Transparency Statement|Sitemap|About US| Contact US: help@patsnap.com