Breast ultrasound image tumor segmentation method based on full convolution network
An ultrasound image and breast tumor technology, which is applied in the field of breast ultrasound image tumor segmentation based on a fully convolutional network, can solve the problems of blurred boundaries, many network parameters, and takes a long time, and achieves the effect of convenient training and high learning rate.
- Summary
- Abstract
- Description
- Claims
- Application Information
AI Technical Summary
Problems solved by technology
Method used
Image
Examples
Embodiment Construction
[0056] The actual breast ultrasound image test is carried out on the segmentation method proposed by the present invention. The training set includes 400 breast ultrasound images, which are used to train the fully convolutional network. To evaluate the segmentation accuracy of the proposed method, 170 breast ultrasound images were used for testing, and an experienced sonographer delineated the margins to determine the gold standard for segmentation.
[0057] In order to evaluate the segmentation effect of the DFCN+PBAC algorithm of the present invention, the following five methods are compared: (1) FCN-8s [2] with migration training from the pre-trained VGG-16 network; (2) U-net [3 ]; (3) Dilated Residual Networks (DRN) [8] using dilated convolutions; (4) DFCN without dilated convolutions; (5) DFCN.
[0058] Among the above algorithms, FCN-8s, U-net and DRN are the three state-of-the-art methods, which have been proven to be effective. To evaluate the impact of dilated convo...
PUM
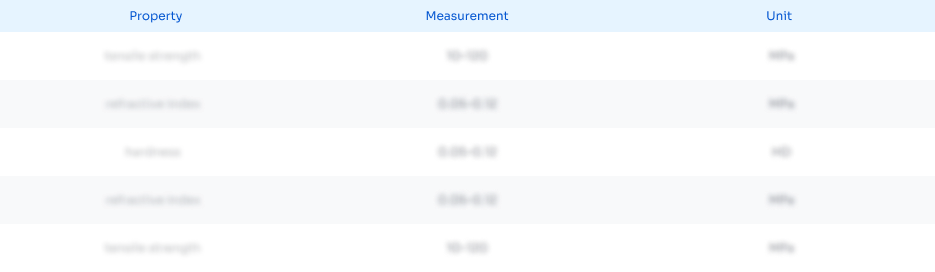
Abstract
Description
Claims
Application Information
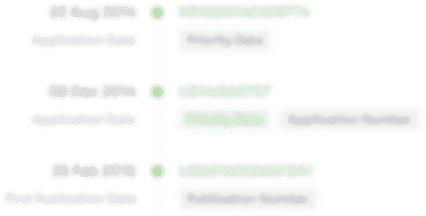
- R&D
- Intellectual Property
- Life Sciences
- Materials
- Tech Scout
- Unparalleled Data Quality
- Higher Quality Content
- 60% Fewer Hallucinations
Browse by: Latest US Patents, China's latest patents, Technical Efficacy Thesaurus, Application Domain, Technology Topic, Popular Technical Reports.
© 2025 PatSnap. All rights reserved.Legal|Privacy policy|Modern Slavery Act Transparency Statement|Sitemap|About US| Contact US: help@patsnap.com