Chinese image semantic description method combined with multilayer GRU based on residual error connection Inception network
A semantic description, network technology, applied in neural learning methods, biological neural network models, instruments, etc., can solve the problems of sentence coherence and readability, and low recognition rate.
- Summary
- Abstract
- Description
- Claims
- Application Information
AI Technical Summary
Problems solved by technology
Method used
Image
Examples
Embodiment Construction
[0052] The specific embodiments of the present invention will be further described in detail below in conjunction with the accompanying drawings.
[0053] A Chinese image semantic description method based on a residual connection-based Inception network combined with a multi-layer GRU network is a Chinese image semantic description method based on the Inception-residual module combined with a multi-layer GRU network; the Inception-residual module is a pair of Inception_v3 (GoogleNet series The third version of the deep convolutional network) The core component of the network, the Inception Architecture module, combines the residual ideas proposed by the residual neural network (ResNet) to design and generate a brand new core component of the deep convolutional network, which makes the network structure While further deepening, the performance of the network will not be degraded, so that deeper features can be extracted. The present invention adopts the deep convolutional neura...
PUM
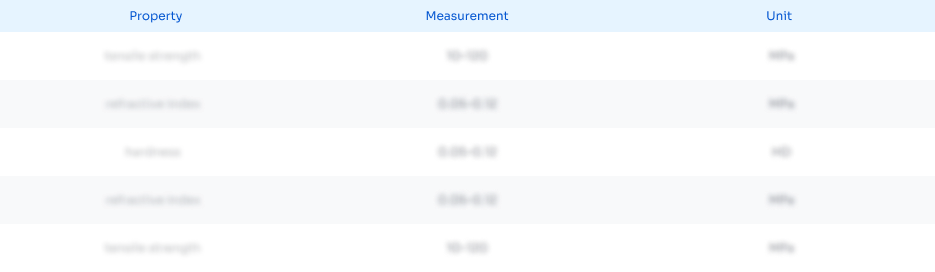
Abstract
Description
Claims
Application Information
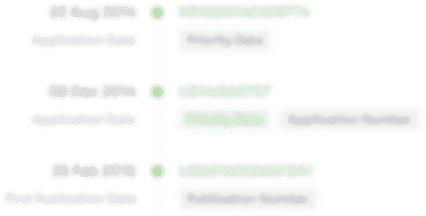
- R&D
- Intellectual Property
- Life Sciences
- Materials
- Tech Scout
- Unparalleled Data Quality
- Higher Quality Content
- 60% Fewer Hallucinations
Browse by: Latest US Patents, China's latest patents, Technical Efficacy Thesaurus, Application Domain, Technology Topic, Popular Technical Reports.
© 2025 PatSnap. All rights reserved.Legal|Privacy policy|Modern Slavery Act Transparency Statement|Sitemap|About US| Contact US: help@patsnap.com