Emotion analysis method based on regional CNN-LSTM
A sentiment analysis and regional technology, applied in special data processing applications, instruments, biological neural network models, etc., can solve the problems of missing features, lack of CNN, and inability to "capture", to achieve the effect of assisting reading and improving efficiency
- Summary
- Abstract
- Description
- Claims
- Application Information
AI Technical Summary
Problems solved by technology
Method used
Image
Examples
experiment example
[0079] We set up several sets of comparative experiments and compare their results with the results of this model. The first set of comparative experiments are two dictionary index-based methods, namely weighted arithmetic mean (wMA) and weighted geometric mean (wGM). The second group is two methods that use regression, which are mean regression (AVG) and maximum regression (MVR). At the same time, we will also use CNN, RNN and LSTM to train and predict separately, and compare the results with the results of this model.
[0080] Evaluation indicators:
[0081] The performance of different methods is mainly measured by the following three indicators
[0082] Mean Squared Error (RMSE):
[0083]
[0084] Mean Absolute Error (MAE):
[0085]
[0086] Pearson correlation coefficient (r):
[0087]
[0088] Among them, A i represents the real value, P i represents the predicted value, and Represents the average value of the true value and the predicted value, respe...
PUM
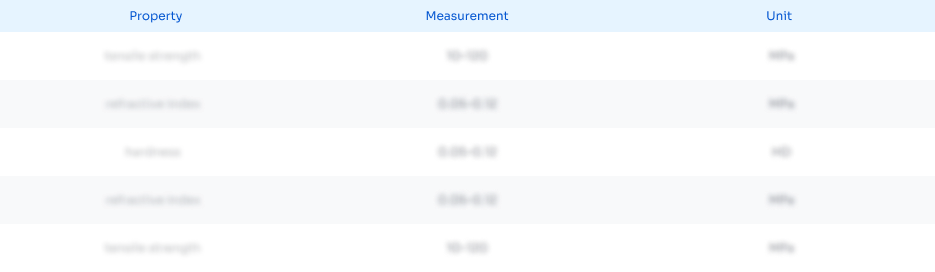
Abstract
Description
Claims
Application Information
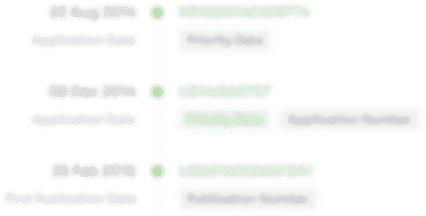
- R&D
- Intellectual Property
- Life Sciences
- Materials
- Tech Scout
- Unparalleled Data Quality
- Higher Quality Content
- 60% Fewer Hallucinations
Browse by: Latest US Patents, China's latest patents, Technical Efficacy Thesaurus, Application Domain, Technology Topic, Popular Technical Reports.
© 2025 PatSnap. All rights reserved.Legal|Privacy policy|Modern Slavery Act Transparency Statement|Sitemap|About US| Contact US: help@patsnap.com