Image quality classification method based on dual-channel deep parallel convolutional network
A convolutional network and image quality technology, applied in the field of image processing, can solve problems such as limited effect of image quality features, failure to fully consider the impact of image semantic information on image quality, and low classification accuracy, so as to improve classification performance and reduce complexity. Sexuality, the effect of eliminating influence
- Summary
- Abstract
- Description
- Claims
- Application Information
AI Technical Summary
Problems solved by technology
Method used
Image
Examples
Embodiment 1
[0028] Since manual design of features requires a lot of experience and relevant background knowledge, it is difficult to manually design image quality features, and the designed features are relatively one-sided, and the classification effect is limited. However, the existing deep learning models do not fully consider the impact of image semantic information on image quality. The classification accuracy is not high. The present invention analyzes and studies these problems, and proposes an image quality classification method based on a dual-channel deep parallel convolution network, see figure 1 , including the following steps:
[0029] (1) Select image samples: Select all images in the database related to image quality evaluation as experimental data, that is, image samples. There are M types of images with different contents in the database, and each type of image contains high-quality image samples and low-quality image samples.
[0030] The image sample data used in the...
Embodiment 2
[0043] The image quality classification method based on dual-channel depth parallel convolution network is the same as embodiment 1, and the construction of dual-channel depth parallel convolution network described in step (3) includes the following steps:
[0044] (3a) Build a seven-layer single-channel deep convolutional network: the single-channel deep convolutional network does not have a parallel convolutional structure, see figure 2 , the specific structure is:
[0045] The first four layers are sequentially connected convolutional layers, the last three layers are sequentially connected fully connected layers, and the fourth convolutional layer is connected to the fifth fully connected layer. Specifically: the first layer is a convolution layer that includes convolution processing, pooling processing, and local response normalization processing; the second layer is a convolution layer that includes convolution processing, pooling processing, and local response normaliz...
Embodiment 3
[0056] The image quality classification method based on dual-channel deep parallel convolutional network is the same as embodiment 1-2, and the two data preprocessing methods described in step (4) and step (6) refer to:
[0057] The first preprocessing method first adjusts the size of the training data set or the image to be tested, scales the original size of the training data set or the image to be tested to a 256*256 size image, and then randomly crops from the scaled image An image block with a size of 227*227 is output as the input image of the first channel of the dual-channel deep parallel convolutional network.
[0058] In the second preprocessing method, an image block with a size of 227*227 is randomly cropped directly from the original size training data set or the image to be tested, and used as the input image of the second channel of the dual-channel deep parallel convolutional network.
[0059] The purpose of randomly cropping image blocks in the present inventi...
PUM
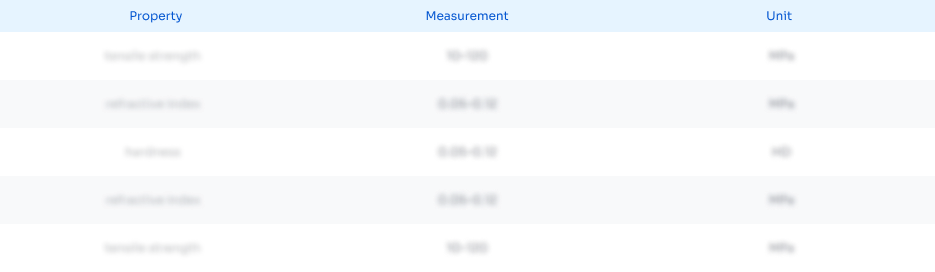
Abstract
Description
Claims
Application Information
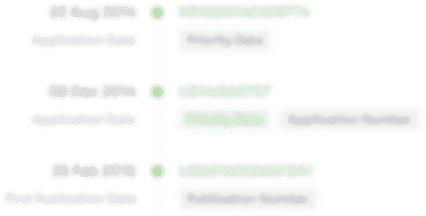
- R&D Engineer
- R&D Manager
- IP Professional
- Industry Leading Data Capabilities
- Powerful AI technology
- Patent DNA Extraction
Browse by: Latest US Patents, China's latest patents, Technical Efficacy Thesaurus, Application Domain, Technology Topic.
© 2024 PatSnap. All rights reserved.Legal|Privacy policy|Modern Slavery Act Transparency Statement|Sitemap