Vehicle GNSS/INS integrated navigation method based on discrete gray neural network model
A gray neural network and integrated navigation technology, applied in the field of vehicle GNSS/INS integrated navigation based on discrete gray neural network model, can solve the problem of reduced navigation accuracy
- Summary
- Abstract
- Description
- Claims
- Application Information
AI Technical Summary
Problems solved by technology
Method used
Image
Examples
Embodiment Construction
[0076] The technical solution of the present invention will be further introduced below in combination with specific implementation methods and accompanying drawings.
[0077] This specific embodiment discloses a vehicle GNSS / INS integrated navigation method based on a discrete gray neural network model, comprising the following steps:
[0078] S1: According to the angular increment and specific force output by the micro-inertial device, use the inertial navigation value update algorithm to solve the attitude, speed and position of the vehicle;
[0079] S2: Establish a discrete gray scale prediction model based on DGM(1,1);
[0080] S3: Improved multi-layer neural network MLP;
[0081] S4: Design a hybrid intelligent prediction algorithm DGM-MLP based on a discrete gray-scale neural network. When the GNSS signal is valid, use DGM-MLP to train the GNSS position; when the GNSS signal is invalid, use DGM-MLP to train the GNSS position. Prediction, obtain pseudo GNSS position in...
PUM
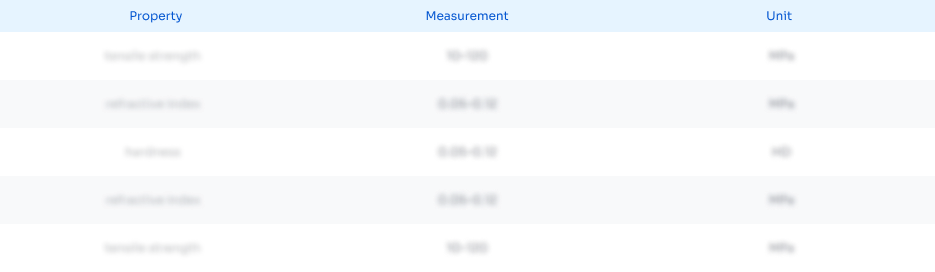
Abstract
Description
Claims
Application Information
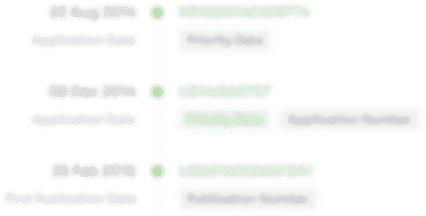
- Generate Ideas
- Intellectual Property
- Life Sciences
- Materials
- Tech Scout
- Unparalleled Data Quality
- Higher Quality Content
- 60% Fewer Hallucinations
Browse by: Latest US Patents, China's latest patents, Technical Efficacy Thesaurus, Application Domain, Technology Topic, Popular Technical Reports.
© 2025 PatSnap. All rights reserved.Legal|Privacy policy|Modern Slavery Act Transparency Statement|Sitemap|About US| Contact US: help@patsnap.com