A method for identifying crop leaf diseases based on improved convolution neural network model AlexNet
A convolutional neural network and disease identification technology, which is applied in the field of crop leaf disease identification based on the improved convolutional neural network model AlexNet, can solve the problems of poor robustness and poor recognition effect, and achieve the effect of improving the recognition effect
- Summary
- Abstract
- Description
- Claims
- Application Information
AI Technical Summary
Problems solved by technology
Method used
Image
Examples
Embodiment Construction
[0026] The present invention is described in further detail in conjunction with accompanying drawing and specific embodiment:
[0027] A crop leaf disease identification method based on the improved convolutional neural network model AlexNet. It is characterized in that it comprises the following steps:
[0028] (1) Acquisition and processing of experimental data;
[0029] (2) Construct a convolutional neural network model;
[0030] (3) Train the convolutional neural network and test the results;
[0031] Described process (1) specifically comprises the following steps:
[0032] The number of data sets needs to be considered when selecting test samples. If the number of data sets is too small, the network will be undertrained and overfitting will occur. Overlearning with a small number of samples will make the model very sensitive to image distortion; if If the sample of the data set is too large, the training speed of the network will be very slow, and the convergence of ...
PUM
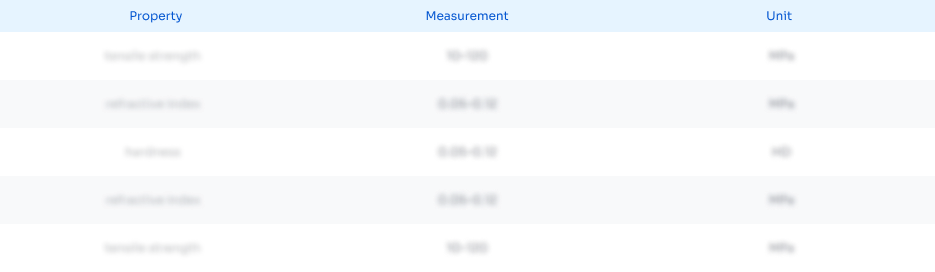
Abstract
Description
Claims
Application Information
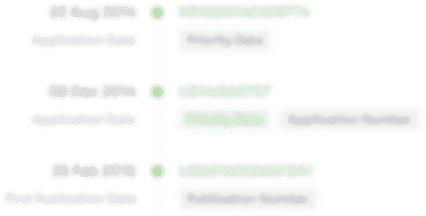
- R&D Engineer
- R&D Manager
- IP Professional
- Industry Leading Data Capabilities
- Powerful AI technology
- Patent DNA Extraction
Browse by: Latest US Patents, China's latest patents, Technical Efficacy Thesaurus, Application Domain, Technology Topic.
© 2024 PatSnap. All rights reserved.Legal|Privacy policy|Modern Slavery Act Transparency Statement|Sitemap