A train condition on-line prediction method based on long-short-time memory network
A long-short-term memory and train state technology, applied in neural learning methods, biological neural network models, railway car body parts, etc., can solve problems such as high fault false alarm rate, inaccurate train state prediction, and low prediction accuracy
- Summary
- Abstract
- Description
- Claims
- Application Information
AI Technical Summary
Problems solved by technology
Method used
Image
Examples
Embodiment Construction
[0078] The present invention will be described in detail below in conjunction with the accompanying drawings and specific embodiments.
[0079] The present invention is based on a long-short-term memory network train state online prediction method, such as figure 1 As shown, the specific steps are as follows:
[0080] Step 1, train status monitoring data preprocessing and normalization, as follows:
[0081] Data preprocessing includes complementing missing data and eliminating mutation points for train status monitoring data;
[0082] Data normalization adopts linear function normalization, removes the dimension of the state monitoring data, limits the data to a certain range, and makes the operation more convenient, as follows:
[0083]
[0084] Among them, x is the monitoring data of the train status at different times, and x min and x max are the minimum and maximum values in the condition monitoring data respectively, x norm It is the status data after normalizat...
PUM
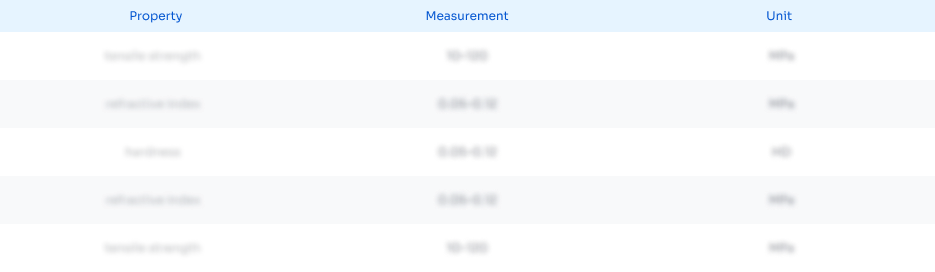
Abstract
Description
Claims
Application Information
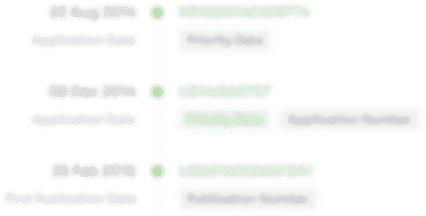
- R&D Engineer
- R&D Manager
- IP Professional
- Industry Leading Data Capabilities
- Powerful AI technology
- Patent DNA Extraction
Browse by: Latest US Patents, China's latest patents, Technical Efficacy Thesaurus, Application Domain, Technology Topic, Popular Technical Reports.
© 2024 PatSnap. All rights reserved.Legal|Privacy policy|Modern Slavery Act Transparency Statement|Sitemap|About US| Contact US: help@patsnap.com