Variation-limited Boltzmann machine based denoising audio feature extraction algorithm
A technology of limited Boltzmann machine and noisy Boltzmann machine, applied in the field of denoising frequency feature extraction algorithm, can solve problems such as time-consuming, and achieve the effect of simple theory
- Summary
- Abstract
- Description
- Claims
- Application Information
AI Technical Summary
Problems solved by technology
Method used
Image
Examples
Embodiment Construction
[0055] The present invention will be further described below in conjunction with the accompanying drawings and embodiments.
[0056] Such as Figure 1-2 Shown, a kind of classification method based on audio characteristic signal, its specific steps are as follows:
[0057] (1) Audio signal collection: collect audio signals and obtain audio samples.
[0058] (2) Signal preprocessing: group the collected audio signals into training and testing groups.
[0059] (3) Construction of the restricted denoising Boltzmann machine model: the learning model is constructed using the excellent unsupervised learning ability of the restricted Boltzmann machine, and the model is divided into a visible layer, a hidden layer and a label layer.
[0060] (4) Denoising audio feature model training: first, the probability of occurrence of audio features is always greater than that of noise features to realize the hidden layer of the pre-trained restricted denoising Boltzmann machine model is divid...
PUM
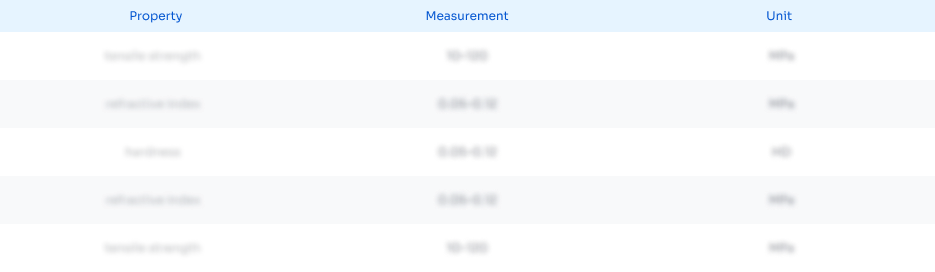
Abstract
Description
Claims
Application Information
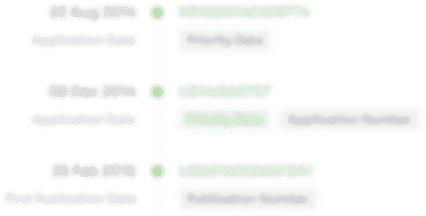
- R&D
- Intellectual Property
- Life Sciences
- Materials
- Tech Scout
- Unparalleled Data Quality
- Higher Quality Content
- 60% Fewer Hallucinations
Browse by: Latest US Patents, China's latest patents, Technical Efficacy Thesaurus, Application Domain, Technology Topic, Popular Technical Reports.
© 2025 PatSnap. All rights reserved.Legal|Privacy policy|Modern Slavery Act Transparency Statement|Sitemap|About US| Contact US: help@patsnap.com